The ModUlar energy system Simulation Environment (MUSE)
Short overview
MUSE is a modelling environment for the assessment of how national or multi-regional energy systems might change over time. Its scope is the entire energy system, from production of primary resources such as oil or biomass, through conversion of these resources into forms of energy for final consumption, and finally the end-use consumption of that energy to meet economy-wide service demands.
MUSE is an agent-based framework, in that it explicitly characterises the decision-making process of firms and consumers in the energy system, thereby capturing a variety of features of market imperfection. MUSE is also technology-rich, in that it characterises the cost and performance of each technology option, tracks technology stock, and provides details on investment, operating costs, energy consumption, and emissions with a detailed bottom-up perspective.
The agent-based modular structure of the sectors is brought together in a partial equilibrium on the energy system through a market clearing algorithm, which balances supply and demand of each energy commodity. The market clearing algorithm is also able to enforce a carbon budget, which escalates a carbon price until agents in all sectors respond and emissions constraints are met.
MUSE-Global is an implementation of a global model in the MUSE framework, characterising 28-regions of the world, and running over a time horizon of 2010 to 2100. It can be used to explore a variety of questions on how to mitigate climate change given realistic constraints and frictions on system change, and has been applied to show that slightly sub-optimal transition pathways may be more likely to succeed than optimal ones.
Key features of the MUSE model
Geographic coverage
The MUSE-Global model covers the entire world, via twenty-eight regions, as shown in the following table:
Geographic region | Countries |
---|---|
AE | Switzerland, Turkey |
ASEAN | Brunei, Cambodia, Indonesia, Lao PDR, Malaysia, Myanmar, Philippines, Singapore, Thailand, Vietnam |
ATE | Armenia, Azerbaijan, Georgia, Kazakhstan, Kyrgyzstan, Tajikistan, Turkmenistan, Uzbekistan |
AUS | Australia, New Zealand |
BRA | Brazil |
CAN | Canada |
CHL | Chile |
CHN | China, Hong Kong, Taiwan |
DNK | Denmark |
EA | Afghanistan, Bangladesh, Bhutan, Chinese Taipei, Cook Islands, Dem. Rep. Korea, East Timor, Fiji, French Polynesia, Kiribati, Macau, Maldives, Mongolia, Nepal, New Caledonia, Pakistan, Papua New Guinea, Samoa, Solomon Islands, Sri Lanka, Tonga, Vanuatu |
ELA | Antigua and Barbuda, Argentina, Aruba, Bahamas, Barbados, Belize, Bermuda, Bolivia, British Virgin Islands, Cayman Islands, Colombia, Costa Rica, Cuba, Dominica, Dominican Republic, Ecuador, El Salvador, Falkland Islands, French Guyana, Grenada, Guadeloupe, Guatemala, Guyana, Haiti, Honduras, Jamaica, Martinique, Montserrat, Netherlands Antilles, Nicaragua, Panama, Paraguay, Peru, Puerto Rico, Saint Lucia, Saint Pierre et Miquelon, St. Kitts and Nevis, St. Vincent and the Grenadines, Suriname, Trinidad and Tobago, Turks and Caicos Islands, Uruguay, Venezuela |
EU7 | Bulgaria, Croatia, Cyprus, Latvia, Lithuania, Malta, N. Cyprus, Romania |
EU18 | Austria, Belgium, Czech Republic, Estonia, France, Germany, Greece, Hungary, Ireland, Italy, Luxembourg, Netherlands, Poland, Portugal, Slovakia, Slovenia, Spain, United Kingdom, Greenland |
FIN | Finland |
IND | India |
ISL | Iceland |
ISR | Israel |
JPN | Japan |
KOR | South Korea |
MEA | Bahrain, Iran, Iraq, Jordan, Kuwait, Lebanon, Oman, Qatar, Saudi Arabia, Syria, United Arab Emirates, Yemen |
MEX | Mexico |
NCA | Algeria, Angola, Benin, Botswana, Burkina Faso, Burundi, Cameroon, Cape Verde, Central African Republic, Chad, Comoros, Congo, Cote d’Ivoire, Dem. Rep. Congo, Djibouti, Egypt, Eq. Guinea, Eritrea, Ethiopia, Gabon, Gambia, Ghana, Guinea, Guinea-Bissau, Kenya, Lesotho, Liberia, Libya, Madagascar, Malawi, Mali, Mauritania, Mauritius, Morocco, Mozambique, Namibia, Niger, Nigeria, Reunion, Rwanda, Sao Tome and Principe, Senegal, Seychelles, Sierra Leone, Somalia, Sudan, Swaziland, Tanzania, Togo, Tunisia, Uganda, Zambia, Zimbabwe |
NOR | Norway |
OETE | Albania, Belarus, Bosnia and Herzegovina, Gibraltar, Kosovo, North Macedonia, Moldova, Montenegro, Serbia, Ukraine |
RUS | Russia |
SWE | Sweden |
USA | United States of America |
ZAF | South Africa |
Energy sectoral detail
As described above, MUSE characterises agent-based decision making in each sector of the economy. The sector breakdown is shown in the following figure:
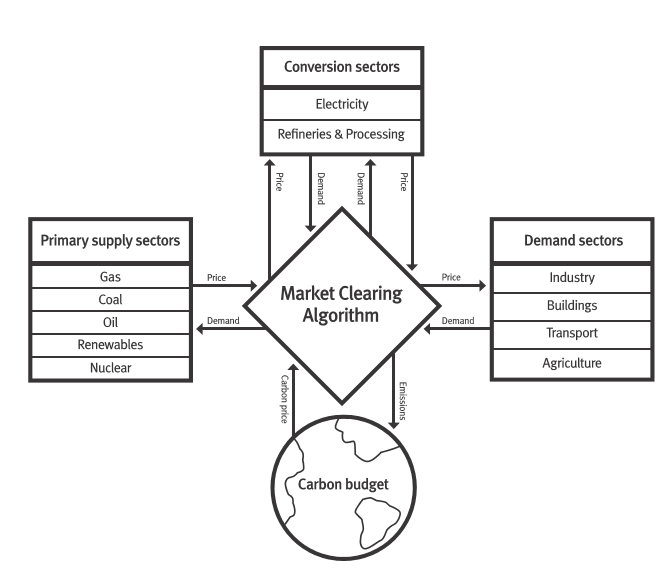
Representation of the MUSE energy sectors and clearing function
Multi-year time periods
The time horizon over which MUSE simulates the evolution of the energy system is divided into a user-chosen number of time periods. In MUSE-Global, to be used in PARIS REINFORCE, there is a starting period of 2010, with further years divided in ten-year periods up to 2100. All years in a given period are considered identical.
Intra-year time periods (time slices)
In addition to the multi-year time periods described above, in MUSE there are time divisions within a year, called “time slices”, which may be defined by the user, so as to capture different resource supply, weather and energy demand conditions at different times of the year. This is the case for instance when the demand for an electricity fluctuates across the year and a variety of technologies may be chosen for its production at given times of the year (such as wind power when wind resources are high, and solar photovoltaics when there is a high availability of solar radiation).
Emissions granularity
As described above, the achievement of climate change targets in MUSE-Global is dealt with via the imposition of emissions limits on each time period. The model tracks the three main sources of greenhouse gases: carbon dioxide (CO2), methane (CH4) and nitrous oxide (N2O). These gases are tracked for each technology, sector, region and for the world, in each time period.
Socioeconomic dimensions
Economic growth
Economic growth is based on the 2nd Shared Socioeconomic Pathway (SSP2), sometimes called the “middle-of-the-road” scenario, as it generally continues recent past trends in demographic, social, economic and lifestyle developments (Fricko et al., 2017) (see the figure below).
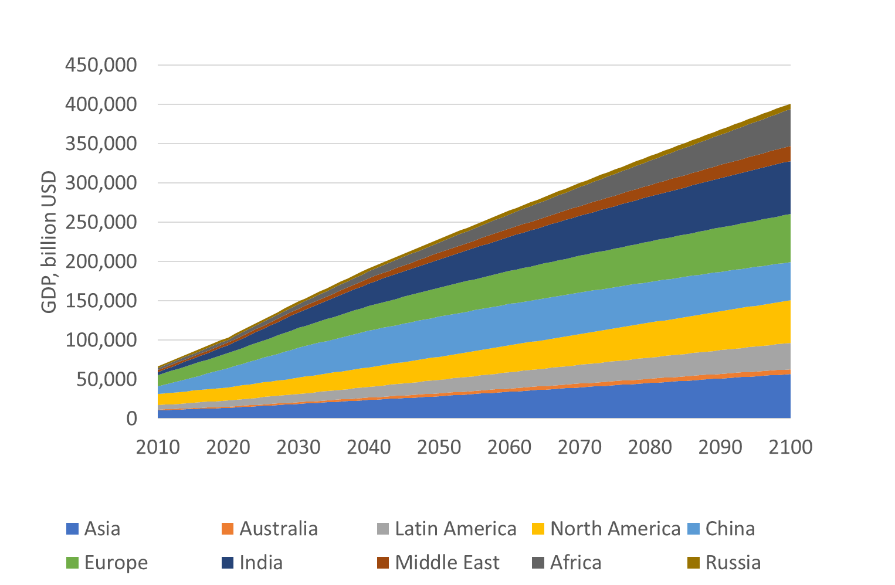
GDP projections in MUSE
Population growth
Population growth is also based on SSP2, with global population peaking to around 9.5 billion by 2050 and dropping back to 9 billion by 2100, as shown in the following figure.
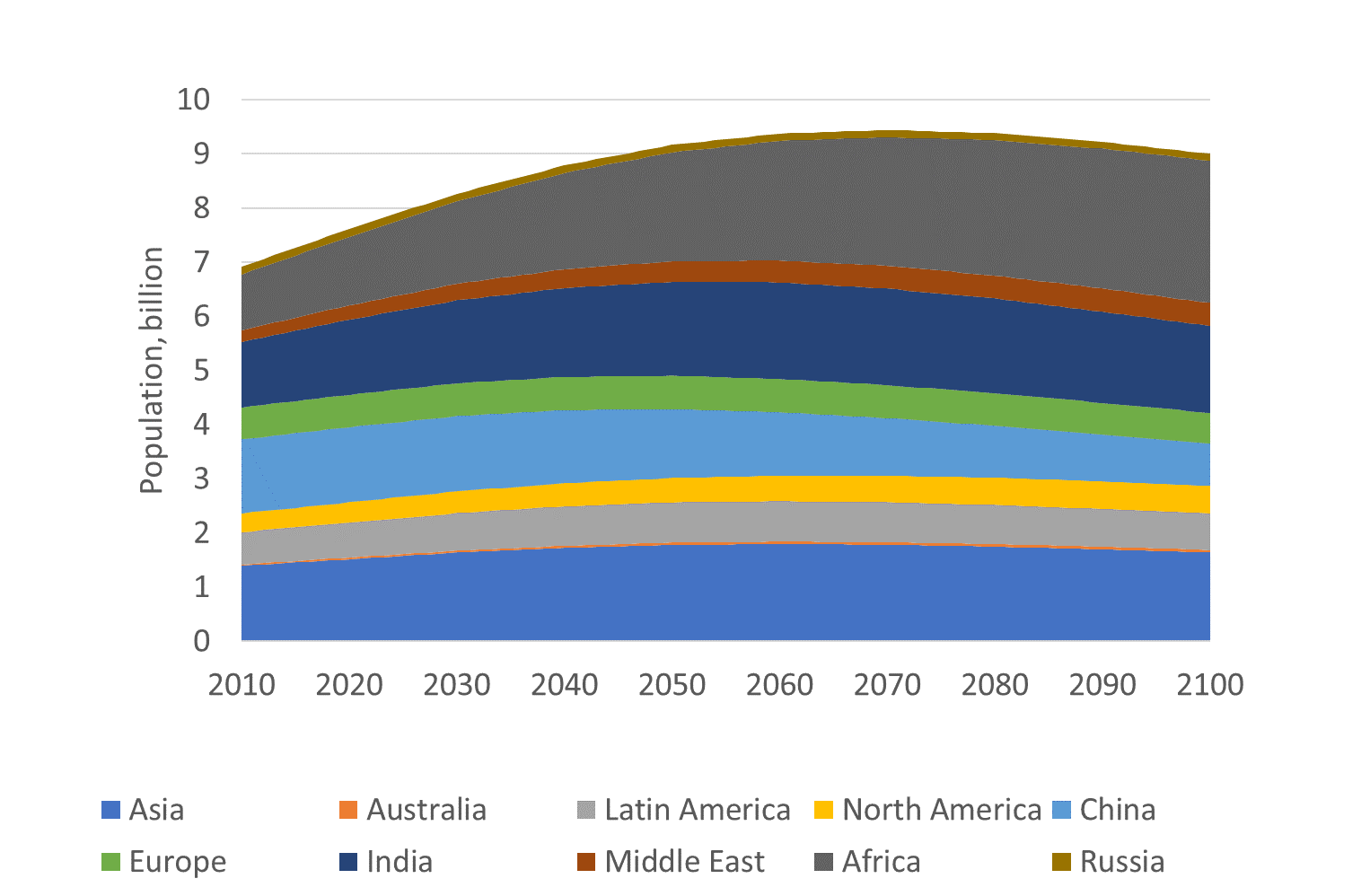
Population projections in MUSE
Sectoral growth
The growth in the industrial, agricultural and retail business sectors in each region is derived from the region’s overall GDP growth, with regression applied to determine historical relationships, and the best fit matches for each service demand category being applied to project growth forwards. With reference to the agricultural sector, the historical trend of service demands (crop and meat demands between 1970 and 2015) are regressed against the exogenously given macroeconomic drivers (GDP and population) (see the figure below, from Kerdan et al., 2019d).
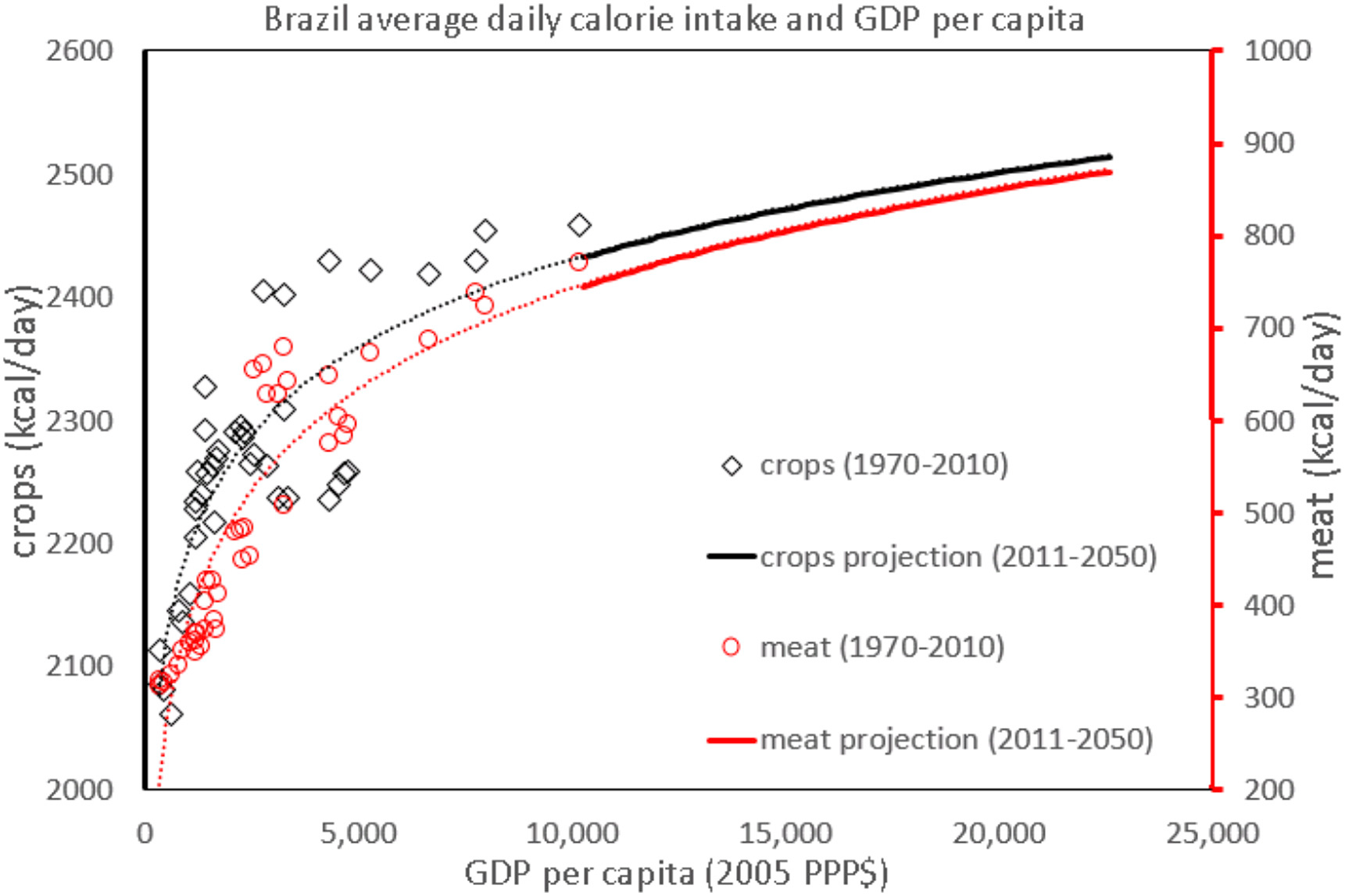
Example of service demand regression over historical macroeconomic drivers in MUSE
Source:Source: Kerdan et al., 2019d
Energy demand drivers
The economic, population and sectoral growths are used to determine specific drivers for the growth in energy demands in a “reference” scenario in which there is no climate change mitigation. For example, in the agricultural sector, demand for one of the services is linked to demand for food. Being highly correlated with income and population growth, the regression model used in MUSE is represented in the following equation, because of the best fit with historical demand of food:
log C = a + b * ln(GDPpc)
where C represents the consumption of food per capita and GDPpc is the region GDP divided over the population.
Energy demand elasticities
MUSE does not include endogenous own-price elasticity. Instead, demand changes may be modelled via sensitivity analysis.
Other socioeconomic dimensions
As described above, MUSE uses an agent-based formulation to determine possible pathways of energy system transition. As such, socioeconomic categorisation of populations of investors and/or consumers is used to capture heterogeneity in investment decision making. The technique relies upon survey data, and widely available socioeconomic metrics, e.g. from the World Bank to achieve this. For example, in the building sector the SINUS-Milieus Typology (Sinus Sociovision, 2017) was used for the classification and attributes assignment of agents. According to the methodology introduced above, socioeconomic survey data of household representative persons can be adopted to allocate households into social groups.
Calibration of the model
The MUSE model is calibrated for the initial period (2010) using IEA world energy statistics for the year 2010, with the projections for energy, installed technology capacity and emissions further calibrated to 2015. The main variables to be calibrated are the capacities and operating levels of all technologies present in the world in 2010; the extracted, exported, imported, produced, and consumed quantities for all energy carriers; and the emissions.
Mitigation/adaptation measures and technologies
MUSE is a technology-rich model that represents most major fossil fuel and low-carbon technologies that are envisaged to be available for at least the first half of the 21st century. As described above, the calibration process determines what technologies existed in the global energy system in the base year. The principal energy sector CO2 mitigation technology options are as shown in the following table:
Upstream | |
---|---|
Synthetic fuel production | Hydrogen production |
Coal to gas with CCS Coal to liquids with CCS Gas to liquids with CCS Biomass to liquids (with and without CCS) |
Electrolysis Coal to hydrogen with CCS Gas to hydrogen with CCS Biomass to hydrogen with CCS |
Electricity | |
Electricity generation | Electricity generation from variable renewables |
Coal with CCS Gas with CCS Nuclear (fission) Hydro (small and large) Biomass (with and without CCS) Geothermal Storage |
Solar PV (ground and rooftop) Concentrated solar power Wind (onshore and offshore) Tidal |
Transport | |
Road | Rail |
Gas (LNG / CNG) vehicles Hybrid/Plugin hybrid electric vehicles (using mixtures of biofuels) Fully electric vehicles Hydrogen fuel cell vehicles Hybrid/Plugin hybrid hydrogen fuel cell vehicles Liquid hydrogen vehicles Flexible vehicles (using biofuel mixtures) |
Electric Hydrogen |
Air | Shipping |
Biofuels in fuel mix Hybrid electric planes Hybrid electric planes using biofuels |
Gas/LNG Hydrogen Biofuels |
Buildings | |
Heating | Lighting and appliances |
Gas (replacing coal/oil)/biomass/electricity/hydrogen boiler Gas/biomass/electricity/hydrogen boilers integrated with solar thermal systems Gas/biomass co-generation (CHP and micro CHP) District heating (gas, biomass, waste heat) standalone or integrated with thermal systems and heat pumps Efficiency |
Efficiency LED lighting systems |
Cooking | Cooling |
Biomass Electricity Hydrogen |
Heat pumps Heat pumps integrated with solar thermal Efficient air conditioning systems |
Industry | |
Iron/steel, pulp/paper, chemicals, aluminum, cement industries | CCS |
Gas replacing oil / coal Biomass Biomethane Electrolysis (for ammonia production) Efficiency |
CCS in iron and steel (with/o bioenergy) CCS in cement (with/o bioenergy) CCS in chemicals (with/o bioenergy) CCS in pulp and paper (with/o bioenergy) CCS in aluminum (with/o bioenergy) |
Agriculture |
|
Energy | non CO2 emissions |
Gas replacing fuel oil Biomass Electricity Mechanisation |
Mechanisation |
Economic rationale and model solution
MUSE simulates a microeconomic equilibrium on the energy system. It consists of modular independent agent-based sector modules, joined together by a market clearing algorithm.
The market clearing algorithm iterates across all of the sector modules, interchanging price and quantity of each energy commodity in each region, until an equilibrium is reached. It sends commodity prices to the end-use sectors and receives back demand for each of these commodities. It sums up these demands and sends them to the conversion and/or supply sectors, which in turn send back a price. This is used to inform an updated price in the market clearing algorithm, whence the procedure iterates again (i.e. updated prices are sent to the end-use sectors, etc). Eventually this process results in a microeconomic equilibrium for each energy commodity in each region. When investigating climate change mitigation, a carbon budget is imposed on each time period. A GHG emissions price is then set in the market clearing algorithm such that the carbon budget is achieved (i.e. by pricing emissions, and thereby incentivising investment in low emissions technology in all sectors via the agent-based modelling described below).
Each agent-based sector module in MUSE is modelled in a way that is appropriate for that sector (i.e. MUSE is a hybrid modular approach). As such, MUSE developers are able to characterise investment decision making in different ways in each sector, to capture the features that are the most important and therefore produce a more realistic representation of energy system transitions. MUSE uses socioeconomic and firm-level data and analyses to characterise a set of investment decision makers (agents) for each sector. Each sector then applies an agent-based modelling (ABM) approach where “agents” (firms or consumers) apply rules to (a) determine which technologies will be considered for investment; (b) calculate a set of objectives according to their decision making preferences; and (c) use a method to combine these objectives to make a final investment decision. Each of these steps is bespoke, where developers can choose from a set of pre-defined rules or can code and add their own objectives and decision rules.
As such, overall MUSE is a limited-foresight model that strives to represent the frictions and challenges that could occur as the world aims for systemic technology change to achieve climate change mitigation over the coming eight decades. Data paucity, technology cost and performance uncertainty, and spatial and temporal coarseness are all weaknesses of this approach, as they are with any other form of integrated assessment modelling.
Key parameters
The MUSE model requires inputs concerning the degree to which energy demand, as well as demand for other goods and services which result in GHG emissions (such as agricultural demand), will change over the time horizon. Future demand projections of each service in each region are based on societal input variables, i.e. population and GDP.
Policy questions and SDGs
Key policies that can be addressed
-
MUSE is usually applied by specifying either a carbon tax or a carbon emissions constraint
for the world as a whole. This acts as a proxy for all other climate-related policies.
However, a further range of policy levers are possible to implement in MUSE, as follows:
- Capacity factor limits on fossil fuel power generation plants (e.g. to simulate minimum or maximum desired levels of operation)
- Subsidies on particular technologies (through adjusting their costs)
- Constraints on the availability of particular technologies (e.g. “no nuclear”, variable renewables accounting for no more than 50% of electricity generation, etc.)
- Constraints on the growth rates of particular technologies (e.g. CCS power generation capacity cannot grow at more than 20% per year), addition of capacity (e.g. cannot grow more than 5 GW per year, and cumulative capacity limits (e.g. cannot exceed 60 GW in total, ever).
Implications for other SDGs
MUSE does not calculate non-climate SDGs. However, it is possible to take MUSE’s outputs and perform “off-model” calculations to estimate many of the SDG implications. For example, MUSE reports the quantity of offshore wind power plants in each of its reporting years. This allows an estimation of the employment that such activity would generate in the region. Furthermore, MUSE may be coupled with macroeconomic and/or lifecycle assessment tools in order to calculate SDG-relevant quantities; for example, impact on income distribution, impact on air or water quality, etc.
Model presentation
Download slides in pdfRecent use cases
Paper | Topic | Key findings |
---|---|---|
Kerdan et al. (2019a) | Assessing gas infrastructure pathways for the southern states of Brazil | Results suggest that, due to the expected increase in regional gas demand in South Brazil, the existing gas infrastructure would require additional investments. Depending on the renegotiation outcomes between Brazil and Bolivia (i.e. either maintaining constant, halving, or halting the Bolivian import of gas), natural gas demand could be covered by a share of alternative supply options, such as an increase in pre-salt production, LNG imports and imports from a new Argentinian pipeline. |
Kerdan et al. (2019b) | Exploring the complex relationship between sugarcane production, deforestation and fossil fuel resource exploitation under two 2°C scenarios for Brazil obtained by either limiting the natural gas or the bioenergy supply | Results suggest that that the promotion of bioenergy in Brazil, should be accompanied by strong policies on limiting deforestation which still represents an important source of emissions. On the other hand, emissions from natural gas can be compensated by the capture and sequestration potential of the Brazilian forests as the natural gas supply helps lowering the deforestation rates. In this context where bioenergy supply reduces, new investments would be necessary to boost the existing gas infrastructure capacity. |
Kerdan et al. (2019c) | An analysis focused on carbon sequestration in Brazil comparing reforestation and sugarcane expansion on abandoned agricultural lan | The results suggest that should Brazil enforce policies on promoting reforestation, it would have the potential to become a large GHG abatement region thanks due to its high carbon (C) sequestration rates. Brazil is expected to liberate up to 68.4 Mha of agricultural land by 2050. If this land is abandoned, the country carbon stock could be reduced from 135.9 PgC in 2010 to 129.9 PgC. If a sugarcane expansion policy is followed, by mid-century the carbon stock could reach 134.2 PgC, whereas if a reforestation policy is implemented it could reach 139.2 PgC. |
Kerdan et al. (2019d) | An analysis exploring the role of land use and reforestation in achieving carbon mitigation targets in Brazil | The model tracks agricultural technology diffusion, energy use, agrochemical demands and its implication on land use and energy and non-energy emissions. Results show the importance of reforestation as a significant contributor to carbon sequestration. Brazil has the potential to sequester around 5.6 GtCO2 by 2050 through reforestation. In this scenario, the capital investment in carbon sequestration and storage would be substantially reduced. |
Sachs et al. (2019a) | Capturing many of the characteristics of the consumers’ behaviour affecting investment decisions | The paper sets out the MUSE agent-based method for modelling of the investment decision making processes of heterogeneous decision makers in the energy system. The integration of several decision-making steps including information gathering, the assessment of the performance of each option as well as the final selection enables a more flexible and realistic representation of the energy system change compared to the majority of the energy systems models. The agent-based method leads to a range of technologies in the market during a transition phase, continuous investment in low capital cost technologies, and eventually the emergence of a low carbon system based on new mass market technologies. |
Sachs et al. (2019b) | Spatially- and temporally-resolved estimation of global space heating, space cooling and hot water demand | The result is the first self-consistent analysis of three energy end-uses (global space heating, space cooling, and hot water demand) at a global scale, disaggregated into country and energy density categories, and provided in profile form to capture seasonal and diurnal demand variations. Global space heating demand reflect not only population and temperature characteristics but also the correlation of gross domestic product with the ownership of air conditioning equipment. In terms of energy density, the results show that a relatively small portion of demand (approx. ∼ 5%) occurs at very high energy density locations (i.e. above 36.9 GW h/km2), while ~50% of demand occurs in low energy density locations (i.e. below 1.79 GW h/km2). |
Crow et al. (2018) | Modelling dynamic supply curves | The paper presents how dynamic upstream gas supply curves are modelled using a global, bottom-up model of the natural gas supply. In contrast to most “static” supply-side models, which bracket resources by average cost, the MUSE upstream gas model creates a range of dynamic outputs by simulating investment and operating decisions in the upstream gas industry triggered in response to forward price and/or demand signals.c |
References
Fricko, O., Havlik, P., Rogelj, J., Klimont, Z., Gusti, M., Johnson, N., ... & Ermolieva, T. (2017). The marker quantification of the Shared Socioeconomic Pathway 2: A middle-of-the-road scenario for the 21st century. Global Environmental Change, 42, 251-267.
Kerdan, I. G., Giarola, S., & Hawkes, A. (2019). A novel energy systems model to explore the role of land use and reforestation in achieving carbon mitigation targets: A Brazil case study. Journal of Cleaner Production.
Kerdan, I. G., Giarola, S., Jalil-Vega, F., & Hawkes, A. (2019). Carbon Sequestration Potential from Large-Scale Reforestation and Sugarcane Expansion on Abandoned Agricultural Lands in Brazil. Polytechnica, 1-17.
Kerdan, I. G., Jalil-Vega, F., Toole, J., Gulati, S., Giarola, S., & Hawkes, A. (2019). Modelling cost-effective pathways for natural gas infrastructure: A southern Brazil case study. Applied Energy, 255, 113799.
Kerdan, I.G., Giarola, S., & Hawkes, A. (2019). Implications of Future Natural Gas Demand on Sugarcane Production, Land Use Change and Related Emissions in Brazil, J. sustain. dev. energy water environ. syst.
Sachs, J., Meng, Y., Giarola, S., & Hawkes, A. (2019a). An agent-based model for energy investment decisions in the residential sector. Energy, 172, 752-768.
Sachs, J., Moya, D., Giarola, S., & Hawkes, A. (2019b). Clustered spatially and temporally resolved global heat and cooling energy demand in the residential sector. Applied Energy, 250, 48-62.
Crow, D. J., Giarola, S., & Hawkes, A. D. (2018). A dynamic model of global natural gas supply. Applied energy, 218, 452-469.