The Global Change Assessment Model (GCAM v2022)
Short overview
The Global Change Assessment Model (GCAM v2022) is a global integrated assessment model that represents both human and Earth system dynamics. It explores the behaviour and interactions between the energy system, agriculture and land use, the economy and climate. The role of GCAM is to bring multiple human and physical Earth systems together in one place to provide scientific insights that would not be available from the exploration of individual scientific research lines. The model components provide a faithful representation of the best current scientific understanding of underlying behaviour.
GCAM allows users to explore what-if scenarios, quantifying the implications of possible future conditions. These outputs are not predictions of the future; they are a way of analysing the potential impacts of different assumptions about future conditions. GCAM reads in external “scenario assumptions” about key drivers (e.g., population, economic activity, technology, and policies) and then assesses the implications of these assumptions on key scientific or decision-relevant outcomes (e.g., commodity prices, energy use, land use, water use, emissions, and concentrations).
It is used to explore and map the implications of uncertainty in key input assumptions and parameters into implied distributions of outputs, such as GHG emissions, energy use, energy prices, and trade patterns. Techniques include scenarios analysis, sensitivity analysis, and Monte Carlo simulations.
GCAM has been used to produce scenarios for national and international assessments ranging from the very first IPCC scenarios (Response Strategies Working Group, 1990) through the present Shared Socioeconomic Pathways (SSPs) (Calvin et al., 2017).
Key features of the GCAM v2022 model
GCAM takes in a set of assumptions and then processes those assumptions to create a full scenario of prices, energy and other transformations, and commodity and other flows across regions and into the future. The energy, agriculture and land use, economy and climate systems are interconnected and interact with each other (see the figure below). The interactions between these different systems are modelled as one integrated whole.
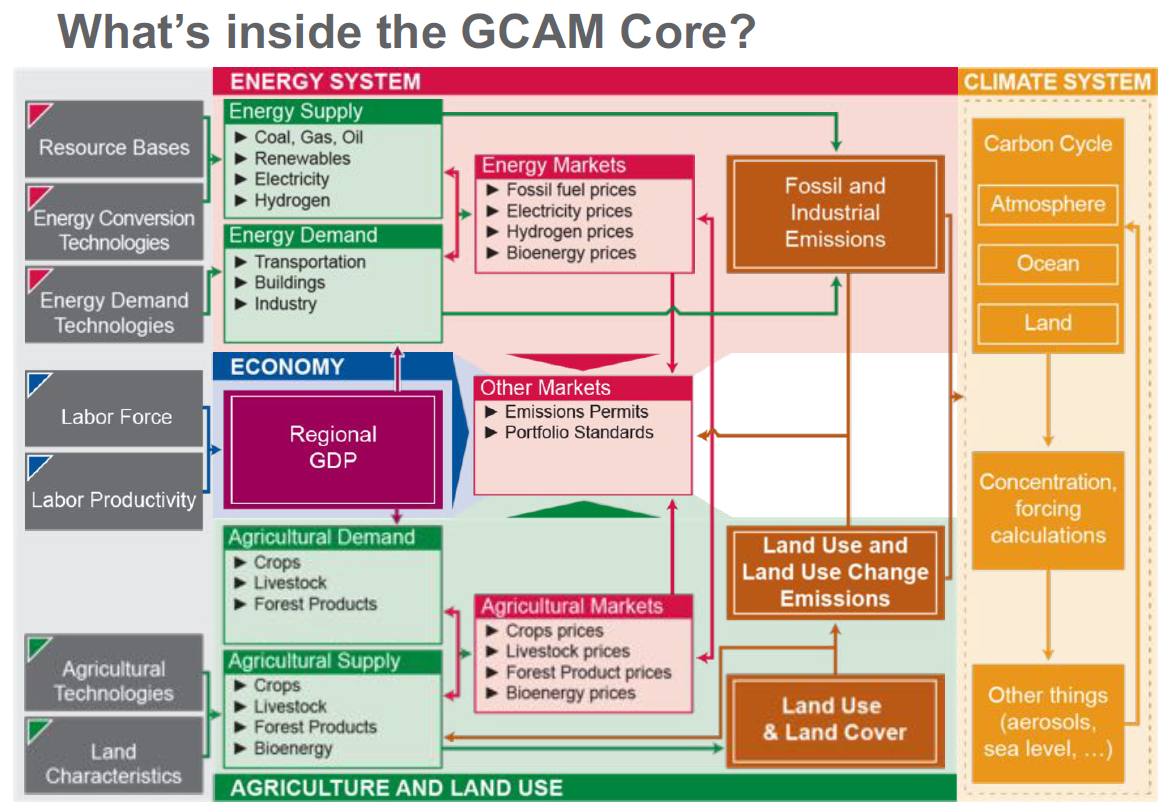
Representation of GCAM v2022 Core functioning
Geographic coverage
The GCAM core represents the entire world, but it is constructed with different levels of resolution for each of these different systems. The energy-economy system operates at 32 geopolitical regions globally , water withdrawals are tracked for 235 hydrologic basins worldwide, and to avoid overlap between geopolitical regions and hydrologic basins, agriculture and land use is calibrated for 384 regions worldwide.
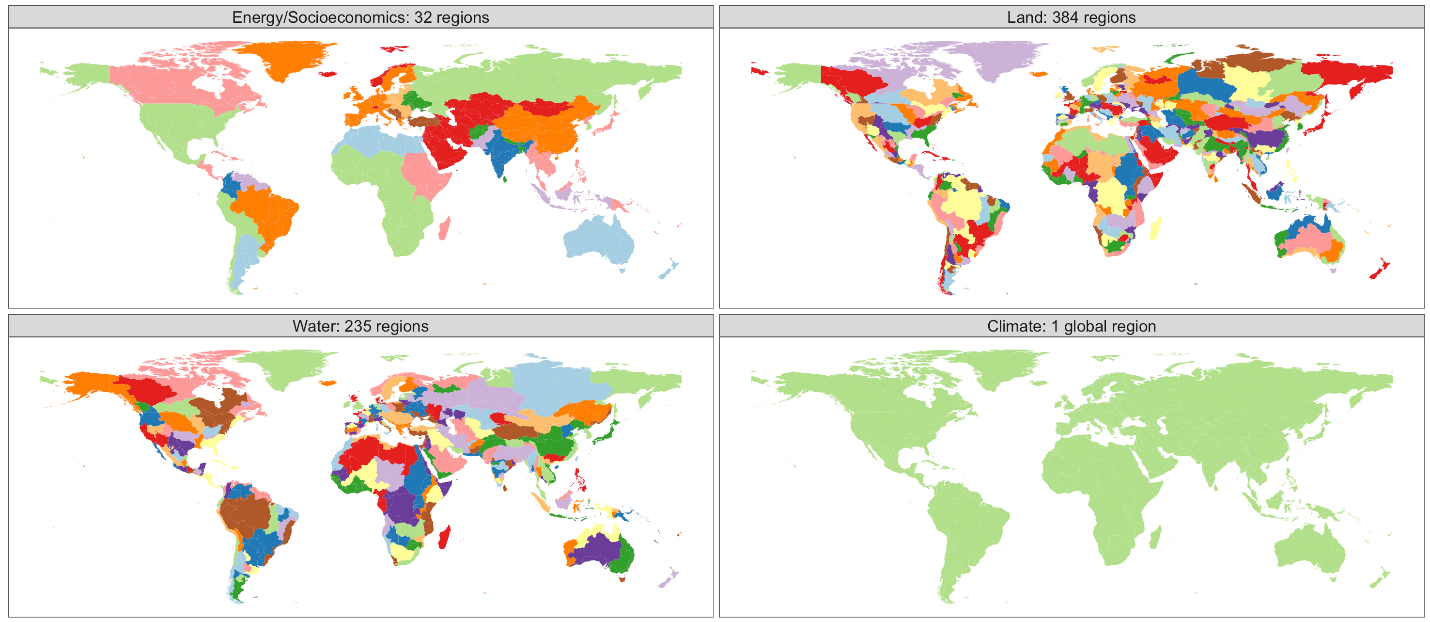
GCAM v2022 regional mapping
Geographic region | Countries |
---|---|
Eastern Africa | Burundi, Comoros, Djibouti, Eritrea, Ethiopia, Kenya, Madagascar, Mauritius, Reunion, Rwanda, Sudan, Somalia, Uganda |
Northern Africa | Algeria, Egypt, Western Sahara, Libya, Morocco, Tunisia |
Southern Africa | Angola, Botswana, Lesotho, Mozambique, Malawi, Namibia, Swaziland, Tanzania, Zambia, Zimbabwe |
Western Africa | Benin, Burkina Faso, Central African Republic, Cote d’Ivoire, Cameroon, Democratic Republic of the Congo, Congo, Cape Verde, Gabon, Ghana, Guinea, Gambia, Guinea-Bissau, Equatorial Guinea, Liberia, Mali, Mauritania, Niger, Nigeria, Senegal, Sierra Leone, Sao Tome and Principe, Chad, Togo |
Argentina | Argentina |
Australia and New Zealand | Australia, New Zealand |
Brazil | Brazil |
Canada | Canada |
Central America and the Caribbean | Aruba, Anguilla, Netherlands Antilles, Antigua & Barbuda, Bahamas, Belize, Bermuda, Barbados, Costa Rica, Cuba, Cayman Islands, Dominica, Dominican Republic, Guadeloupe, Grenada, Guatemala, Honduras, Haiti, Jamaica, Saint Kitts and Nevis, Saint Lucia, Montserrat, Martinique, Nicaragua, Panama, El Salvador, Trinidad and Tobago, Saint Vincent and the Grenadines |
Central Asia | Armenia, Azerbaijan, Georgia, Kazakhstan, Kyrgyzstan, Mongolia, Tajikistan, Turkmenistan, Uzbekistan |
China | China |
Colombia | Colombia |
EU-12 | Bulgaria, Cyprus, Czech Republic, Estonia, Hungary, Lithuania, Latvia, Malta, Poland, Romania, Slovakia, Slovenia |
EU-15 | Andorra, Austria, Belgium, Denmark, Finland, France, Germany, Greece, Greenland, Ireland, Italy, Luxembourg, Monaco, Netherlands, Portugal, Sweden, Spain, United Kingdom |
Eastern Europe | Belarus, Moldova, Ukraine |
European Free Trade Association | Iceland, Norway, Switzerland |
Non-EU Europe | Albania, Bosnia and Herzegovina, Croatia, Macedonia, Montenegro, Serbia, Turkey |
India | India |
Indonesia | Indonesia |
Japan | Japan |
Mexico | Mexico |
Middle East | United Arab Emirates, Bahrain, Iran, Iraq, Israel, Jordan, Kuwait, Lebanon, Oman, Palestine, Qatar, Saudi Arabia, Syria, Yemen |
Pakistan | Pakistan |
Russia | Russia |
South Africa | South Africa |
Northern South America | French Guiana, Guyana, Suriname, Venezuela |
Southern South America | Bolivia, Chile, Ecuador, Peru, Paraguay, Uruguay |
Southeast Asia | American Samoa, Brunei Darussalam, Cocos (Keeling) Islands, Cook Islands, Christmas Island, Fiji, Federated States of Micronesia, Guam, Cambodia, Kiribati, Lao Peoples Democratic Republic, Marshall Islands, Myanmar, Northern Mariana Islands, Malaysia, Mayotte, New Caledonia, Norfolk Island, Niue, Nauru, Pacific Islands Trust Territory, Pitcairn Islands, Philippines, Palau, Papua New Guinea, Democratic People’s Republic of Korea, French Polynesia, Singapore, Solomon Islands, Seychelles, Thailand, Tokelau, Timor Leste, Tonga, Tuvalu, Viet Nam, Vanuatu, Samoa |
South Korea | South Korea |
Taiwan | Taiwan |
USA | United States |
Energy system detail
The structure of the energy system consists of four main elements: resource production, energy transformation, final energy carriers and end-use (see the figure below). It also tracks international trade in energy commodities. All the different elements of GCAM interact through market prices and physical flows of, for example, electricity.
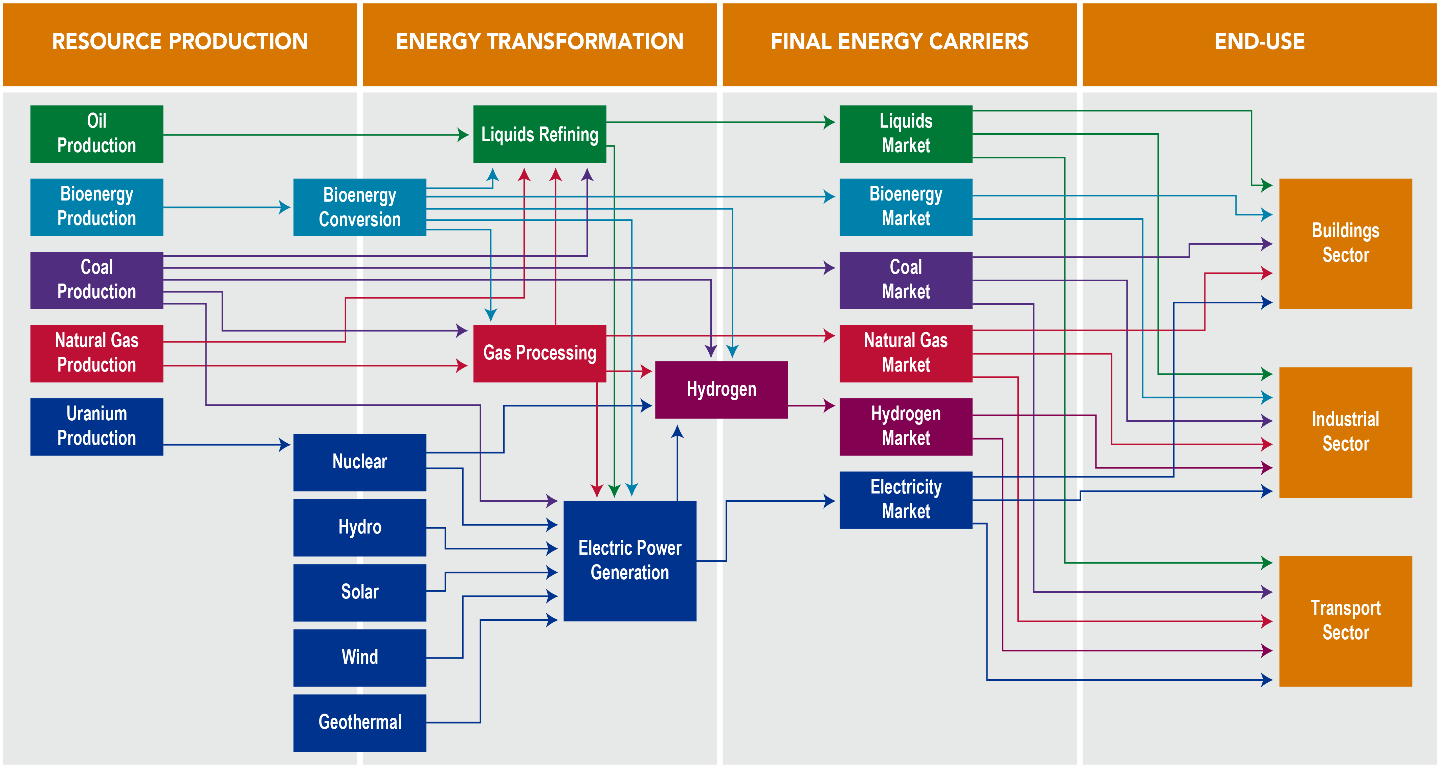
Structure of Energy system in GCAM
Land and agricultural system detail
Within each of the land regions shown in the second figure, land is categorised into approximately a dozen types based on cover and use. In GCAM, competing uses of land are nested within land nodes. Within each land node, it is generally assumed to be easier to substitute products. Among arable land types, further divisions are made for lands historically in non-commercial uses (forests and grasslands) and commercial land uses (commercial forests and croplands). Production of approximately twenty crops is currently modelled, with specific yields depending on the land region and management type (with and without irrigation, high and low fertiliser).
Climate module & emissions granularity
GCAM uses a global climate carbon-cycle climate module, Hector (Calvin et al., 2019), an open-source, object-oriented, reduced-form global climate carbon-cycle model that represents the most critical global-scale earth system processes. At every time step, emissions from GCAM are passed to Hector. Hector converts these emissions to concentrations and calculates the associated radiative forcing and the response of the climate system (e.g., temperature, carbon-fluxes, etc.).
Gas | Sector | Gas | Sector |
---|---|---|---|
C02** | AgLU***, Energy | NH3 | AgLU, Energy |
CH4 | AgLU, Energy, Industrial Processes, Urban Processes | SO2 | AgLU, Energy, Industrial Processes |
N20 | AgLU, Energy, Industrial Processes, Urban Processes | CO | AgLU, Energy, Industrial Processes |
SF6 | Energy, Industrial Processes | BC | AgLU, Energy |
HFCs | Energy, Industrial Processes, Urban Processes | OC | AgLU, Energy |
NOx | AgLU, Energy, Industrial Processes | ||
NMVOC | AgLU, Energy, Industrial Processes, Urban Processes | ||
C2F6 | Energy, Industrial Processes | ||
CF4 | Industrial Processes, Urban Processes |
* Most of these gases also have positive or negative indirect effects on radiative forcing
**CO2 emissions from the AgLU sector are separate from CO2 emissions from the Energy sector. Any change in atmospheric carbon occurs as a function of anthropogenic fossil fuel and industrial emissions, land-use change emissions and the atmosphere-ocean and atmosphere-land carbon fluxes.
***AgLU = Agriculture and Land Use
Hector has a three-part main carbon cycle: a one-pool atmosphere, three-pool land, and four-pool ocean (see the figure below). The atmosphere consists of one well-mixed box. The ocean consists of four boxes, with advection and water mass exchange simulating circulation. The high-latitude surface ocean takes up carbon from the atmosphere, while the low-latitude surface ocean off-gases carbon to the atmosphere. The land consists of a user-defined number of biomes or regions for vegetation, detritus and soil. The vegetation takes up carbon from the atmosphere while the detritus and soil release carbon back into the atmosphere. The earth pool is continually debited with each time step to act as a mass balance check on the carbon system. Hector actively solves the inorganic carbon system in the surface ocean, directly calculating air– sea fluxes of carbon and ocean pH. It reproduces the global historical trends of atmospheric [CO2], radiative forcing, and surface temperatures.
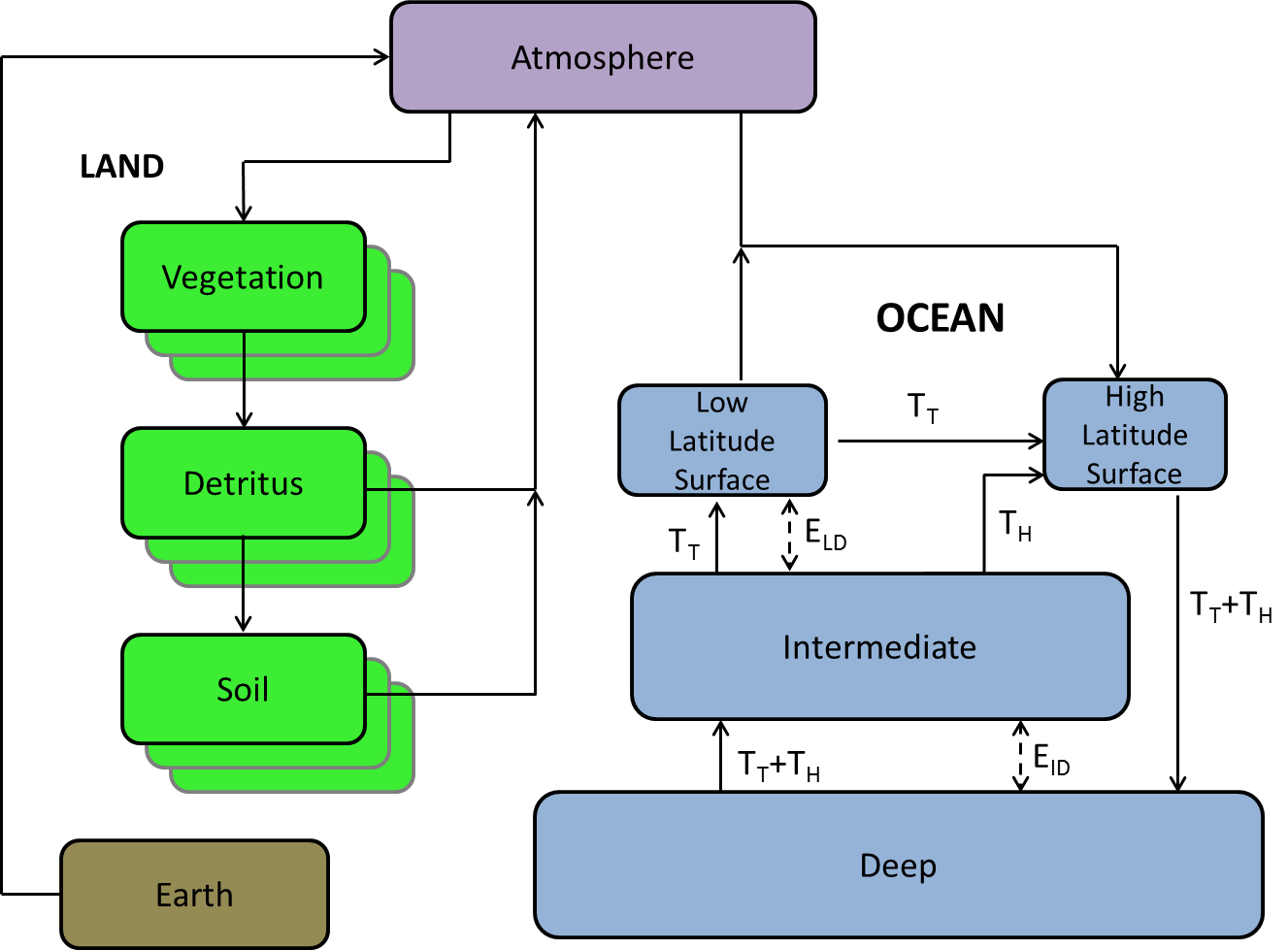
Representation of Hector’s carbon cycle, land, atmosphere, and ocean used in GCAM
Socioeconomic dimensions
Economic growth
The socioeconomic components of GCAM set the scale of economic activity and associated demands for model simulations. Assumptions about population and per capita GDP growth for each of the 32 geo-political regions together determine the GDP. Population and economic activity are used in GCAM to transfer information to other GCAM components, and are determined exogenously.
GCAM’s inputs include information on population and the rate of per capita income growth for each of the energy-economic regions. Each scenario requires assumptions about population and per capita GDP growth for future time periods, for example those of the SSPs. The macro-economic module takes both of these to produce overall GDP in each GCAM energy-economic region, where the regional GDP is calculated using a simple one-equation model.:

Where r stands for region, t for period, t Step = number of years in the time step (5 for GCAM v2022), 〖GDP〗_(r,t) for GDP in region r in period t, 〖POP〗_(r,t) for population in region r in period t and 〖GRO〗_(r,t) for annual average per capita GDP growth rate in region r in period t.
Industrial sector growth
Industrial sector growth Growth in industrial sectors is based on a pre-defined GDP elasticity which decreases over time, thus assuming saturation of industrial demand with rising incomes. The values of these elasticities vary per region and between different SSPs. Apart from a GDP elasticity, industrial demand also depends on an assumed price elasticity for industrial energy use.
Transport sector growth
Per capita demand for passenger transport depends on an assumed GDP per capita elasticity and price elasticity, which means that more transport services are demanded at higher incomes and cheaper transport. However, there is also a time cost involved in transport, which increases proportionally with GDP per capita, and which is summed to the capital and energy costs of transport. This means that, at higher incomes, demand for passenger transport slowly saturates due to the price elasticity multiplied by the increasing costs of time. Also, demand shifts to faster ways of transport, for which time costs are lower. Demand for freight transport, like for industrial sectors, depends on an assumed GDP elasticity and price elasticity.
Building sector growth
Demand for energy services in residential and commercial buildings is primarily by total building floor space, which is driven by GDP per capita, and saturates at a given maximum value, defined per region. Per unit of floor space, demand for energy services increases over time depending on the relative price of the service, and the difference between the base year saturation and the defined maximum saturation for a certain service. For heating and cooling services, the maximum saturation depends on regionally explicit heating and cooling degree days.
Demand for agricultural commodities
Demand for agricultural and forest commodities depend on exogenous income and price elasticities. For per-capita food demand, also elasticities of substitution of substitution are applied, where demand moves from staple to non-staple crops with increasing GDP. All elasticities are assumed equal between model regions, but the projected evolution of food demand per region depends largely on differences in base-year food consumption patterns.
Mitigation/adaptation measures and technologies
GCAM is a technology-rich model that represents most major fossil fuel and low-carbon technologies that are envisaged to be available for at least the first half of the 21st century. By simulating the substitution of low-carbon for high-carbon technologies in response to their relative costs, as well as emissions constraints and/or carbon prices, the GCAM model simulates mitigation through a large set of different measures.
Upstream | |
---|---|
Synthetic fuel production | Hydrogen production |
Coal to gas with CCS Coal to liquids with CCS Gas to liquids with CCS Biomass to liquids (with and without CCS) |
Electrolysis Coal to hydrogen with CCS Gas to hydrogen with CCS Thermal splitting (nuclear) |
Electricity and heat | |
Electricity generation | Heat generation |
Coal with CCS Gas with CCS Nuclear (fission and fusion) Hydro Biomass (with and without CCS) Geothermal Solar PV Solar CSP Wind (onshore) Marine |
Coal with CCS Gas with CCS Oil with CCS Geothermal Biomass Biomass with CCS |
Transport | |
Road | Rail |
Gas (LNG / CNG) vehicle Hybrid electric vehicles Fully electric vehicles Hydrogen fuel cell vehicles Biofuels in fuel mix |
Electric Hydrogen Efficiency |
Air | Marine |
Biofuels in fuel mix |
Gas Hydrogen Biofuels Efficiency |
Buildings | |
Heating | Lighting |
Gas replacing coal / oil Biofuels Electricity Efficiency |
|
Behaviour | Cooling |
Behavioural change |
Electricity |
Process heat | CHP |
Gas replacing oil / coal Biomass Hydrogen Electricity |
Gas replacing oil / coal Biomass |
Land & Animal husbandry | Behaviour |
Land yield maximisation Improved feeding practices |
Behavioural changes (less product demand) |
Land Use, Land Use Change, Forestry | |
Afforestation Land production Biomaterials |
Also, certain assumption sets can be loaded for future crop yields, heating and cooling degree days, which are consistent with a certain temperature target. Through these assumptions, the model adapts to the simulated reality through the allocation of agricultural production, and building service demands.
Economic rationale and model solution
The core operating principle for GCAM is that of market equilibrium. The representative agents in the modules use information on prices and make decisions about the allocation of resources. They represent, for example, regional electricity sectors, regional refining sectors, regional energy demand sectors, and land users who have to allocate land among competing crops within any given land region. Markets are the means by which these representative agents interact with one another. Agents indicate their intended supply and/or demand for goods and services in the markets. GCAM solves for a set of market prices so that supplies and demands are balanced in all these markets across the model (see the figure below); in other words, market equilibrium is assumed to take place in each one of these markets (partial equilibrium), and not in the entire economy across all markets (general equilibrium). The GCAM solution process is the process of iterating on market prices until this equilibrium is reached. Markets exist for physical flows such as electricity or agricultural commodities, but they also can exist for other types of goods and services, for example tradable carbon permits.
As an example, in any single model period, GCAM derives a demand for natural gas starting with all of the uses to which natural gas might be put, such as passenger and freight transport, power generation, hydrogen production, heating, cooling and cooking, fertiliser production, and other industrial energy uses. Those demands depend on the external assumptions about, for example, electricity generating technology efficiencies, but also on the price of all of the commodities in the model. GCAM then calculates the amount of natural gas that suppliers would like to supply given their available technology for extracting resources and the market price. The model gathers this same information for all of the commodities and then adjusts prices so that in every market during that period supplies of everything from rice to solar power match demands.
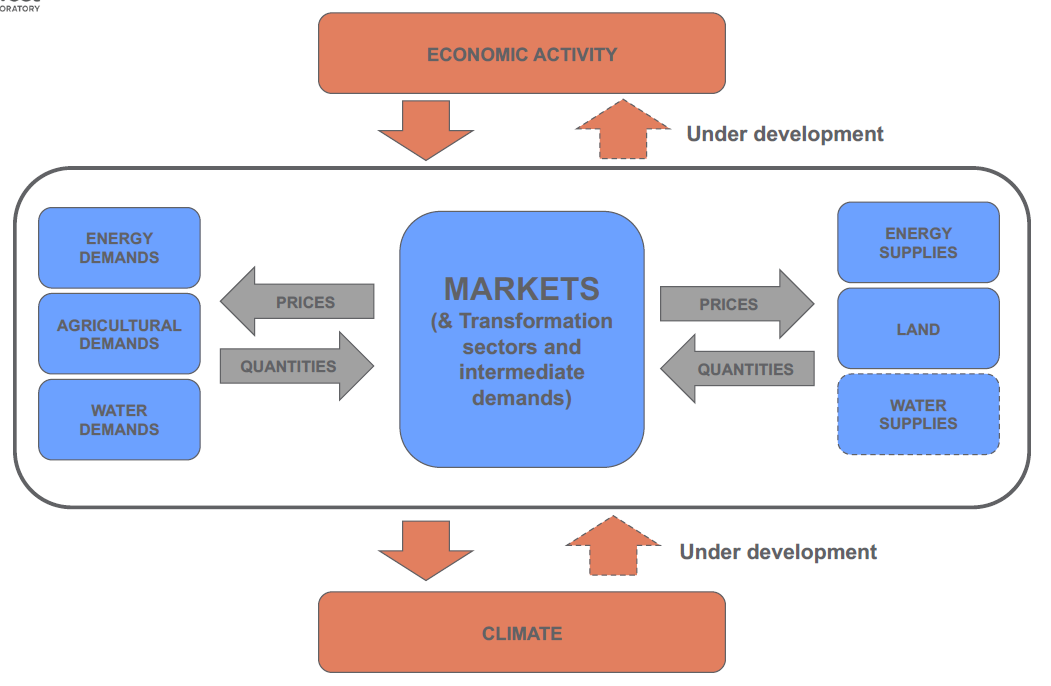
Conceptual Schematic of the Operation of the GCAM Core
GCAM is a dynamic recursive model, meaning that decision-makers do not know the future when making a decision today, as opposed to other optimisation models, which assume that agents know the future with certainty when they make decisions. After it solves each period, the model then uses the resulting state of the world, including the consequences of decisions made in that period—such as resource depletion, capital stock retirements and installations, and changes to the landscape—and then moves to the next time step and performs the same exercise. The GCAM version used is typically operated in five-year time steps with 2100 as the final calibration year. However, the model has flexibility to be operated at a different time horizon through user-defined parameters.
While the agents in the GCAM model are assumed to act towards maximising their own self-interest, the model as a whole is not performing an optimisation calculation. In fact, actors in GCAM can make decisions that “seemed like a good idea at the time”, but which are not optimal from a larger social perspective and which the decision maker would not have made had the decision maker known what lay ahead in the future. For example, the model’s actors do not know about future climate regulations, and could install fossil fuel power in the years preceding the implementation of such policies. Also, stated preferences by agents for certain technologies (e.g. transport modes) are calculated in the base year and such preferences are persistent into the future (their evolution can be changed by the user), preventing the solution to be completely cost-minimising or profit-maximising.
Key parameters
Key scenario assumptions for the GCAM core include socioeconomics (population, labour participation, and labour productivity); energy technology characteristics (e.g. costs, performance, water requirements); agricultural technology characteristics (e.g. crop yields, costs, carbon contents, water requirements, fertiliser requirements); energy and other resources, such as fossil fuels, wind, solar, uranium and groundwater; and policies, including emissions constraints, renewable portfolio standards, etc.
Key scenario results (outputs) from the GCAM model include an analysis of the energy system (energy demands, flows, technology deployments, and prices throughout); prices and supplies of all agricultural and forest products, land use and land use change; water demands and supplies for all agricultural, energy, and household uses; and emissions for 24 greenhouse gases and short-lived species (CO2, CH4, N2O, halocarbons, carbonaceous aerosols, reactive gases, and sulphur dioxide).
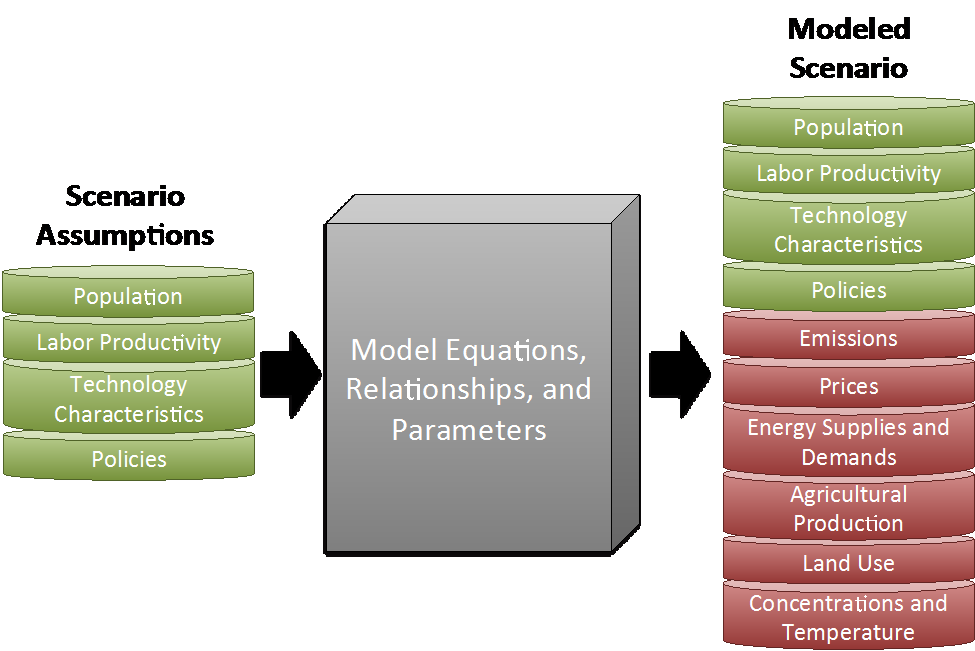
GCAM scenario assumptions and modelling outputs
Outcomes in GCAM depend strongly on the assumptions made for socioeconomic, techno-economic, and agronomic parameters. While many of these parameters matter for the outcomes from the model, some can be identified as the most relevant ones:
• Global and national GHG reduction targets • Global and national afforestation targets • Future demand scenarios for energy services and agricultural products • Future cost paths for renewable energy and CCS technologies
Parameters can be revised and updated in the framework of the PARIS REINFORCE project, following the feedback of local experts (stakeholder engagement), the comparative assessment with other modelling experiences, and the discussion with the partners (modellers).
Policy questions and SDGs
Key policies that can be addressed
One of GCAM’s uses is to explore the implications of different future policies. There are a number of types of policies that can be easily modelled in GCAM:
Emissions-related policies: There are three main policy approaches that can be applied in GCAM to reduce emissions of CO2 or other GHGs: carbon/GHG prices, emissions constraints, or climate constraints. In all cases, GCAM implements the policy approach by placing a price on emissions. This price then filters down through all systems in GCAM and alters production and demand. For example, a price on carbon would put a cost on emitting fossil fuels. This cost would then influence the cost of producing electricity from fossil-fired power plants that emit CO2, which would then influence their relative cost compared to other electricity generating technologies and increase the price of electricity. The increased price of electricity would then make its way to consumers that use electricity, decreasing its competitiveness relative to other fuels and leading to a decrease in electricity demand.
• Carbon or GHG prices: GCAM users can directly specify the price of carbon or GHG emissions. Given a carbon price, the resulting emissions will vary depending on other scenario drivers, such as population, GDP, resources, and technology. • Emissions constraints: GCAM users can specify the total amount of (CO2 or other GHG) emissions as well. GCAM will then calculate the price of carbon needed to reach the constraint in each period of the constraint. • Climate constraints: GCAM users can specify a climate variable (e.g. concentration or radiative forcing) target for a particular year. Users determine whether that target can be exceeded prior to the target year. GCAM will adjust carbon prices in order to find the least-cost path to reaching the target.
Emissions prices of different GHGs can be linked together for a multi-gas policy. Additionally, markets in GCAM can be set for any emission species (e.g., CH4-only market, NOx market, etc.). In addition to identifying emission prices as one measure of cost, GCAM employs the “deadweight loss” approach to measuring welfare loss from emissions mitigation efforts through carbon pricing.
Energy production policies: There are times when users would like to explore the implications of a constraint on production or a minimum production requirement. This capability allows GCAM users to model policies such as renewable portfolio standards and biofuels standards. Across sectors, these constraints must be applied as quantity constraints, but they can be applied as share constraints within individual sectors (e.g. fraction of electricity that comes from solar power). In implementing these policies, users can set either a lower bound or an upper bound. The model will solve for the tax (upper bound) or subsidy (lower bound) required to reach the given constraint.
Land use policies
• Protected Lands: With this policy, GCAM users can set aside a fraction of natural land, removing it from economic competition. This land cannot be converted to crops, pasture, or any other land type. This is similar to real-world policies such as reducing emissions from deforestation and forest degradation (REDD). • Valuing carbon in land: When applying a price on carbon through any of the emissions-related policy approaches, GCAM users can choose whether that price extends to land use change CO2 emissions. This policy is modelled as a subsidy to land-owners for holding carbon stocks as opposed to a price on the emissions themselves. • Bioenergy constraints: GCAM users can impose constraints on bioenergy within GCAM. Under such a policy, GCAM will calculate the tax or subsidy required to ensure that the constraint is met.
Key policies that can be addressed
GCAM does not automatically calculate the implications on non-climate SDGs of its least-cost energy system to meet prescribed climate or emissions constraints. However, it is possible to use its outputs and calculate the predictions for certain indicators framed in the SDG agenda.
SDGs | GCAM v2022 |
---|---|
§2. Zero hunger (e.g., food prices, shortages) | Food prices by region |
§3. Health (e.g., air-pollution related mortality) | Mortality due to air pollutants |
§6. Clean water and sanitation (e.g., groundwater depletion) | Groundwater depletion by river basin |
§7. Affordable and clean energy (e.g., traditional biomass use, %renewable energy) | Traditional biomass use and renewable energy penetration |
§12: Responsible production & consumption (e.g., % recycled waste, embedded emissions) | Footprint impact of consumption |
§15: Life on land (e.g., land use for forests, rate of land use change) | Land use for forests and rate of land use change |
Model presentation
Video
Slides
Download slides in pdfRecent use cases
Paper | Topic | Key Findings |
---|---|---|
(Huang et al., 2019) | Global agricultural green and blue water consumption under future climate and land use changes | Global crop green water consumption will increase by 12% in 2090s when compared with that in 1971–2000, and climate change will dominate over land use change in determining the trend of global crop green water consumption. However, expansion in global irrigated area will dominate the changing trend of global crop blue water consumption which increases 70% by 2090s, especially in regions with significant irrigated land expansion (e.g. northern Africa, central Asia, China, Mexico, the Middle East, Russia, southern Asia, and Argentina). Furthermore, global crop blue water dependence will increase under climate and land use changes, especially in arid regions. |
(Markandya et al., 2018) | Health co-benefits from air pollution and mitigation costs of the Paris Agreement | The health co-benefits substantially outweighed the policy cost of achieving the 2°C target. The ratio of health co-benefit to mitigation cost ranged from 1.4 to 2.45, depending on the scenario. At the regional level, the costs of reducing GHG emissions could be compensated with the health co-benefits alone for China and India, whereas the proportion the co-benefits covered varied but could be substantial in the European Union (7–84%) and USA (10–41%), respectively. Finally, we found that the extra effort of trying to pursue the 1.5°C target instead of the 2°C target would generate a substantial net benefit in India (US$3.28–8.4 trillion) and China ($0.27–2.31 trillion). |
(Fawcett et al., 2015) | Understanding what the INDCs collectively deliver in terms of reducing the probability of the highest levels of global mean surface temperature change and improving the odds of limiting temperature change to under 2°C relative to preindustrial levels | To limit warming to any level, CO2 emissions at the global level must ultimately be brought to zero. If emissions are not brought swiftly to zero beyond 2100, the chances of extreme temperature change after 2100 could be much higher and the chance of limiting warming to 2°C much lower. The Paris scenarios reduce probabilities of extreme warming and increase the probability of limiting global warming to 2°C this century. |
References
Huang, Z., Hejazi, M., Tang, Q., Vernon, C. R., Liu, Y., Chen, M., & Calvin, K. (2019). Global agricultural green and blue water consumption under future climate and land use changes. Journal of Hydrology, 574, 242-256.
Markandya, A., Sampedro, J., Smith, S. J., Van Dingenen, R., Pizarro-Irizar, C., Arto, I., & González-Eguino, M. (2018). Health co-benefits from air pollution and mitigation costs of the Paris Agreement: a modelling study. The Lancet Planetary Health, 2(3), e126-e133.
Fawcett, A. A., Iyer, G. C., Clarke, L. E., Edmonds, J. A., Hultman, N. E., McJeon, H. C., ... & Creason, J. (2015). Can Paris pledges avert severe climate change?. Science, 350(6265), 1168-1169.