MAPLE
Overview
The China-MAPLE model consists of an energy system optimisation module which is based on the TIMES modelling framework. TIMES is a modelling platform for local, national or multi-regional energy systems, which provides a technology-rich basis for estimating how energy system operations will evolve over a long-term, multiple-period time horizon (Loulou and Labriet, 2007). It follows a techno-economic (bottom-up) approach to describe the energy sectors in many details through a variety of specific technologies characterised with their technical and economic parameters. TIMES offers thus a detailed representation of energy sectors, which includes extraction, transformation, distribution, end uses, and trade of various energy forms and materials. TIMES computes an equilibrium on energy markets (partial equilibrium) and determines an optimal configuration of the energy systems to satisfy service demands at a minimum cost over a long-term horizon, while respecting GHG emission limits.
The MAPLE model simulates the investment and operation of major energy technologies under constraints of emissions reductions of GHGs and pollutants in local regions in China. The model can project and simulate future energy use trends in reference scenarios and other comparative scenarios of varying degrees of mitigation action. The calculation objective of the model is that the total cost of the energy system must reach the minimum while exogenously given energy demand and any other major constraints on the energy system (e.g. technology availability and growth rates) are satisfied. The costs include investment costs, residual values of assets, fixed and variable operating costs and maintenance costs, local energy extraction costs, the costs of energy imports beyond China, gains from exports to regions outside of China, major energy transmission and distribution costs, related taxes and additional subsidies.
Key features of the model
Energy sectoral detail
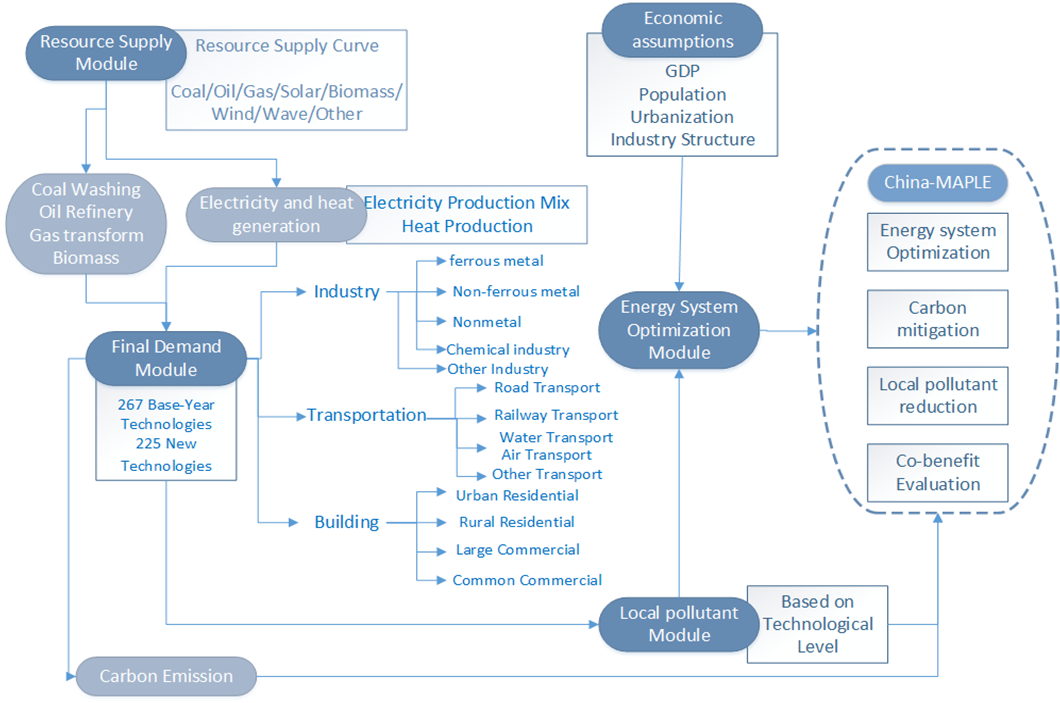
Representation of the China-MAPLE energy system for China national-level analysis
Geographic coverage
The MAPLE version 1.0 model has one region (China), i.e. the analysis is based on the national level. In version 2.0, for the residential sector, the geographic granularity of the model includes 31 provinces (regions). That is because the residential sector accounts for a large amount of particulate matter (PM2.5) emissions, and MAPLE aims to analyse the co-benefits of emissions reductions for air quality. For the most recent version, more regions have been developed for other sectors, including the residential, electricity, and industrial sectors and sub-sectors.
Multi-year time periods
The model performs calculations on five-year steps, from 2010 to 2050, currently being updated to establishing 2015 as the base year.
Intra-year time periods (time slices)
When explicitly referring to the electricity sector, it is possible to include intra-annual time slices by season and day-night, to represent the specific variation in electricity supply (such as from time- and weather-dependent renewables like wind and solar photovoltaics) and demand during different typical periods of the day and year (e.g. winter versus summer, day versus night).
Time-slices are especially important whenever the mode and cost of production of an energy carrier at different times of the year are significantly different (Loulou and Labriet, 2007). This is the case for instance when the demand for an electricity fluctuates across the year and a variety of technologies may be chosen for its production at given times of the year (such as wind power when wind resources are high, and solar photovoltaics when there is a high availability of solar radiation). In such cases, the matching of supply and demand requires that the activities of the technologies producing and consuming the electricity be tracked—and matched—in each time slice.
GHG Emission and local pollutant emissions
In MAPLE 1.0 and 2.0, carbon dioxide (CO2) is considered as the main GHG emission. The other main emissions, including methane (CH4) and nitrous oxide (N2O), are expected to be added in the next version. For the local pollutant emissions, in version 2.0, sulphur dioxide (SO2), nitrogen oxides (NOx), and primary particulate matters are considered as main emissions related to China’s air quality improvement.
Drivers of energy and other GHG-emitting service demands
GDP, population and urbanisation
The general socioeconomic parameters, including GDP growth rate, population, urbanisation rate, and industry structure, are exogenous. The current socioeconomic modelling assumption is consistent with the model of the World Bank (2012) and other literature. The average annual growth rate of the GDP of China will drop to nearly 6.2% in 2020 and will further decrease to approximately 4% after 2030. The model assumes a population growth scenario in which having a second child is publicly allowed. Based on various sources (Zeng et al., 2013), the model assumes that the total population of China will gradually increase from 1.36 billion in 2010 to 1.433 billion by 2020, peak by 2025-2030, and thereafter decrease to 1.385 billion by 2050. The urbanisation rate of China reached 54.77% in 2014; the rate will reach 58.2% in 2020, 67.1% in 2030, and 75.2% in 2050. These assumptions can be varied in light of updated socioeconomic projection information.
Energy demand drivers
In the TIMES modelling framework, the economic, population and sectoral growths are used to determine specific drivers for the growth in energy demands in reference scenarios with no climate change mitigation policy. For example, the demand for the number of billion kilometre-vehicles (BVkm) travelled by automobiles is based on GDP per capita, whereas the growth in the demand for residential space heating is driven by the number of households. Once the drivers for the different energy demands represented by NATEM are determined and quantified, the construction of the reference demand scenario requires computing a set of energy service demands over the horizon. This is done by choosing elasticities of demands to their respective drivers, in each region, using the following general formula:
Demand = Driver Elasticity
So, for example the number of billion vehicle km travelled by automobiles (BVkm) grows by a factor that is the growth in the GDP per capita in a region to the power of a pre-defined elasticity:
BVkm = (GDP/capita) Elasticity
In most cases the elasticities (which vary over time) are less than 1 and decrease over time. For example, an elasticity of 0.8 means that a 10% increase in the growth of GDP per capita in a region would result in an 8% increase in billions of vehicle kilometres driven. Over time this could reduce to a much smaller elasticity, reflecting empirical evidence that demand for energy services such as automobile transport ultimately saturates with rising incomes.
The TIMES modelling framework also has the capability of estimating the price-based response of these energy service demands to the changing conditions of scenarios in which mitigation occurs. For example, if the cost of energy increases as fossil fuels are replaced by renewables, or because fossil-subsidies are reduced, then the demand for energy services could decrease. To do this, TIMES uses another set of inputs, namely the price elasticities of the demands for each energy service considered. The model can then calculate the new demands for these policy cases.
Calibration of the model
Calibration concludes with the base year calibration, which mainly includes the following aspects: (i) total energy consumption and category-based energy consumption of various sectors and sub-sectors; (ii) total energy consumption and category-based energy consumption of third-level sub-sectors; (iii) energy consumption per unit of end-use demand of major divisions; (iv) CO2 emissions; and (v) emissions of conventional pollutants.
Energy consumption of sub-sectors of the industrial sector is derived on the basis of energy consumption statistics given in the year 2010 (2015 in the updated version in progress) from the Energy Balance Sheet and the Industrial Statistics Yearbook. The energy consumption of the third-level sub-sectors in the industrial sector calculated in the model is summarised according to sub-sectors after calibration on the base year and main statistical data.
The main sources of energy demand data is energy balance tables available from China’s energy statistical yearbook. For energy consumption in the industrial sub-sectors and technologies, we used several sources such as China Statistical Yearbook 2006-2011, Industrial Statistical Yearbook 2010-2013, China Steel Statistics 2011, China Chemical Industry Yearbook, China Nonferrous Metals Industry Yearbook, China Energy Statistical Yearbook 2006-2013. We also use technical parameter documents on production lines of major industrial sectors, as well as calculation and collation from related literature. The data for the transport sector is from Research into China's Medium and Long-Term Development Strategy on Traffic and Transportation, Automotive Energy Outlook 2012, China Transport Yearbook, China Bulletin on Motor Vehicles Pollution Prevention, China Energy Statistical Yearbook 2010-2013, as well as data research and calculation based on major literature. For the building sector, the data sources include The Annual Development and Research Report on Building Energy Efficiency in China (2008-2013), as well as the summary and calculation of relevant literature.
Mitigation/adaptation measures and technologies
China is on the critical stage of its energy transformation reform and meeting its NDC target for 2030. As a technology-rich model, and like other bottom-up models, mitigation/adaptation measures and technologies can be reflected by constraints for commodities and processes. Referring to the “five-year plan” series, detailed emission standard and mitigation technologies are reported for each sector, especially for final demand sectors.
Demand for end-use energy services is the basis of analysis of the bottom-up energy model. Useful energy provided by end-use energy equipment satisfying demand for end-use energy for the current year is a prerequisite for analysis of energy system optimisation. The demand for end-use energy services pertaining to the Chinese regional model is classified into four major sectors, namely the industrial sector, the transportation sector, the building sector (commercial/residential sector), and the agricultural sector and others, according to segments.
The main mitigation measures and technologies considered in the model are listed in the following table, and the technologies with an asterisk (*) could be further added in the model, based on the requirements of the PARIS REINFORCE project. The main assumptions and technological data will be revised and updated to be consistent with the current updating government database and other modelling groups in the project.
Upstream |
|
Synthetic fuel production | Synthetic fuel production |
|
|
Electricity and heat |
|
Electricity generation | Heat generation |
|
|
Transport |
|
Road | Rail |
|
Electric high-speed railway |
Air | Marine |
|
|
Buildings (commercial/residential) |
|
Heating | Lighting |
|
Efficiency |
Appliances | Cooling |
Efficiency | Efficiency |
Industry |
|
Chemical | Non-Metallic |
|
|
Ferrous metal | Non-Ferrous metal |
|
|
Process heat | Machine drives |
|
|
Steam | CHP |
|
|
Agriculture |
|
Energy | Other |
Biomass |
-
The differences between China-MAPLE and other bottom-up models for China is mainly
reflected in four aspects:
- China-MAPLE integrates local pollutant control and co-benefit modules into the energy system framework, and models local pollutant emission control measures and technologies in key areas.
- With regard to the local pollutant module, the link between local emissions and energy systems is based on technical rather than activity levels to reflect the mitigation effects of technological advances and structural adjustments. Regarding the benefit evaluation module, it describes the benefits of local pollutants obtained through emissions reduction.
- China-MAPLE introduces energy supply curves in the energy supply module. The supply of coal, oil and natural gas includes both domestic production and imports, avoiding deviations caused by fixed energy costs.
- MAPLE model is currently applied in the World Bank Group project on China’s energy modelling project, with consideration of linking the bottom-up model with a typical top-down computable general equilibrium approach.
Economic rationale and model solution
TIMES is a dynamic least-cost optimisation model, and as such contains three components: an objective function, variables, and constraints. The first component (objective) corresponds to minimising the net total discounted cost (e.g. 3-5% is typically used in deep decarbonisation studies) of the entire energy system. A single optimisation, which searches for the maximal net total surplus, simulates market equilibrium for each commodity (energy, material, demand). Maximising the net total surplus (i.e. the sum of producers’ and consumers’ surpluses) is operationally done by minimising the net total cost of the energy system. The second component (variables) corresponds mainly to future investments and activities of technologies at each time period, amount of energy produced or consumed by technologies, as well as energy imports and exports. An additional output of the model is the implicit price (shadow price) of each energy form, material and emission, as well as the reduced cost of each technology (reduction required to make a technology competitive). The third component (constraints) corresponds to various limits (e.g. amount of energy resources available) and obligations (e.g. energy balances throughout the system, useful energy demand satisfaction) to be respected.
The main model assumptions leading to optimal outputs include future technological developments and the structure of energy markets. On the one hand, technological progress is exogenously assumed, and economic agents have a perfect foresight of this. On the other hand, energy markets are assumed to be under perfect competition. Moreover, TIMES considers only energy markets, and thus equilibrium is not assumed for all markets in the economy. From that perspective, TIMES computes only a partial equilibrium on energy markets.
Key Parameters
-
China is a country with a large difference in energy production and consumption
technologies among provinces. As a bottom-up technology-rich model, a large number
of data and parameter collection processes are required. The main parameters include:
- Technical parameters: efficiency, availability factor, lifetime, stock for base year, etc.
- Economic parameters: investment cost, operation fixed cost, variable cost, fuel cost, etc.
- Emission parameters: emission coefficient for fuels and for specific technologies.
- Policy parameters: new technology for starting year, new investment for technologies, technology share constraints, emission constraints, carbon tax, etc.
Taking the technical parameters of the power sector as an example, power generation technologies mainly include thermal, nuclear and renewable energy power generation technologies. Coal power and natural-gas-based power generation constitutes the main technology of thermal power generation in China. With regard to technical parameters of three typical coal-fired units, power production efficiency of the supercritical unit is approximately 41-42%, coal consumption per unit of power generation being about 310 grams of coal equivalent (gce)/kWh, and that of the ultra-supercritical unit is 45%-47%, coal consumption per unit of power generation standing at about 280 gce/kWh. The natural gas power generation technology in the base year is mainly natural gas-based gas turbine technology and gas-steam combined cycle units. Natural gas in China has the merits of higher efficiency, relatively environmental protection, smaller floor area and shorter construction period compared with coal-fired power generation. Because construction and operating costs of nuclear power plants are relatively high (about three times that of thermal power plants), its power generation cost is higher (about 0.42-0.54 Yuan/kWh on average). Furthermore, power generation technologies involving wind power, solar energy, biomass, small hydropower and other sorts of renewable energy also play an important role in China's power production.
Parameters can be revised and updated in the framework of the PARIS REINFORCE project, following the feedback of national and local experts (stakeholder engagement), the comparative assessment with other modelling experiences, and the discussion with the partners (modellers).
Policy questions and SDGs
The policy questions considered in MAPLE mainly orient on emission mitigation and energy policy instruments. Like most bottom-up energy models, multiple kinds of constraints can be added during the energy system optimisation process. The main policy granularity in the model is listed in the following table, with further explanation.
Emissions mitigation policy instruments |
|
Tax | Full. Feasible for carbon tax (also other emission tax) constraints for a milestone year. |
Emissions target/quota (annual) | Full. Feasible for the annual emission target for CO2 and local pollutant emissions. |
Emissions target/quota (cumulative) | Partial. Possible, provided sufficient historical data. |
Regulations (emissions standards, etc.) | Full. Regulations like emission standards for a specific sector or energy commodity. Constraints for carbon emission intensity can also be added. |
Energy policy instruments |
|
Tax | Full. Feasible for taxes set on fuels, technologies, other emissions, etc. |
Subsidy | Full. Feasible for subsidy set on energy fuels and technologies; energy service final devices, like gas-oven for coal-to-gas heating policy; and full electric vehicles purchasing behaviour. |
Energy mix target | Full. Energy mix targets for both full-economy sector and for specific sector, e.g. electricity generation, transportation, and industry (with sub-sectors). |
Efficiency target | Full. Efficiency target mainly for technologies, e.g. power generation efficiency; and fuel-economy standard upgrade for gasoline vehicles. |
Regulations (thermal regulation in buildings, banning of diesel cars in urban areas, etc.) | Full. Feasible for regulations for specific sector and energy commodity, e.g. limitation of vehicles with low emission standards in transportation sector, and regulations on low-carbon Ammonia production, etc. |
Furthermore, the SDGs considered in the current version model include mainly health and affordable clean energy, with details shown in the following table.
SDGs | Details |
---|---|
§3. Health (e.g., air-pollution related mortality) | In the local pollutant module, the local pollutant emissions are calculated and optimised. The local pollutant emission coefficient is directly linked to the energy processes/technologies, also considering any the end-of-pipe pollutant control measures. Damages to health can be evaluated based on measures of mortality (VSL – value of statistical life) |
§7. Affordable and clean energy (e.g., traditional biomass use, %renewable energy) | Cost-effectiveness analysis for clean energy is included in the model framework, also considering the environmental co-benefits. |
Recent publications
Publication | Topic | Key findings |
---|---|---|
Yang et al. (2018a) | Air quality benefit of China’s mitigation target to peak its emission by 2030 | The model in this paper assesses the co-benefits of carbon mitigation in local pollutant reduction by linking carbon emissions to local air pollutants at the technological level. |
Yang et al. (2018b) | Cost–benefit analysis of China’s Intended Nationally Determined Contributions based on carbon marginal cost curves | Carbon tax is added into China-Multi-Pollutant Abatement Planning and Long-term Benefit Evaluation (China-MAPLE). Several conclusions are drawn from analysis based on MACC and China-MAPLE model. |
Yang et al. (2018c) | The air quality co-benefit of coal control strategy in China | A bottom-up model of China-MAPLE is developed, to analyze the impact of coal control strategies on energy systems and local pollutant reductions. |
Yang et al. (2016) | A scenario analysis of oil and gas consumption in China to 2030 considering the peak CO2 emission constraint | A bottom-up energy system model is built and applied to analyse the fossil fuel consumption and carbon emissions in China up to 2030. |
Yang et al. (2013a) | Incorporating environmental co-benefits into climate policies: A regional study of the cement industry in China | The model of this paper analyses the impacts of incorporating local air quality improvement and environmental co-benefits into the climate policy and mitigation technology assessment of the cement sector in China. |
Yang et al. (2013b) | Quantifying co-benefit potentials in China’s industry sector during its 12th five year | Co-benefits of mitigation measures in key industry sector are evaluated based on and the policy impact is further analysed based on marginal abatement cost during 12th Five Year Plan period. |
Yang et al. (2018d) | Carbon Mitigation Pathway Evaluation and Environmental Benefit Analysis of Mitigation Technologies in China’s Petrochemical Industry | This model evaluates the carbon mitigation effects and environmental co-benefits of mitigation technologies that have been long ignored in China’s petrochemical and chemical industry. |
Yang et al. (2018e) | Provincial-level Evaluation for the environmental health benefits of residential building energy conservation polices | The model of this article evaluates the effect of energy conservation policies and carbon mitigation efforts on reducing health damage in China’s building sector. |
References
Loulou R. & Labriet M. (2007). ETSAP-TIAM: the TIMES integrated assessment model Part I: Model structure. Computational Management Science 5(1): 7–40.
World Bank. (2012). Data – The World Bank. Retrieved September 20, from http://data.worldbank.org/
Yang, X. & Teng, F. (2018a). Air quality benefit of China’s mitigation target to peak its emission by 2030. Climate Policy, 18(1), 99-110.
Yang, X., Teng. F. & Xi, X. (2018b) Cost–benefit analysis of China’s Intended Nationally Determined Contributions based on carbon marginal cost curves. Applied Energy, 227, 415-425.
Yang, X. & Teng, F. (2018c). The air quality co-benefit of coal control strategy in China. Resources, Conservation and Recycling, 129, 373-382.
Yang, X., Xi, X. & Guo, S. Lin, W., & Feng, X. (2018d). Carbon Mitigation Pathway Evaluation and Environmental Benefit Analysis of Mitigation Technologies in China’s Petrochemical and Chemical Industry, Energies,11(12), 3331.
Yang, X., Xi X., Lin, W., et al. (2018e) Evaluation of environmental health benefits of China’s building energy conservation policies: an integrated assessment on national and provincial levels. International Journal of Environmental Health Research, 28(3), 1-25.
Yang, X., Wan. H., Zhang, Q., Zhou, J.-C., & Chen, S.-Y. (2016). A scenario analysis of oil and gas consumption in China to 2030 considering the peak CO2 emission constraint. Petroleum Science, 13(2), 370-383.
Yang, X., Teng, F., & Wang, G. (2013a) Incorporating environmental co-benefits into climate policies: A regional study of the cement industry in China. Applied Energy, 112, 1446-1453.
Yang, X., Teng, F., & Wang, G. (2013b). Quantifying co-benefit potentials in the Chinese cement sector during 12th Five Year Plan: An analysis based on marginal abatement cost with monetized environmental effect. Journal of Cleaner Production, 58, 102–111.
Zeng,Y., Gu, B., Liang,J., & Guo, Z. (2013). China’s fertility policy adjustment and China’s development. Beijing: Social Sciences Academic Press.