The ATOM model
Short overview
ATOM is an agent-based model,which based on the plausibility of its results compared to historical data and observations, simulates the expected effectiveness of various policy schemes on technology adoption (e.g., small-scale solar PV, battery energy storage systems, smart appliances, electric vehicles, etc.) in the residential sector, for the geographical and socioeconomic context under study. Apart from exploring the expected effectiveness of technology adoption under policy schemes of interest, the model allows us to consider and explicitly quantify the uncertainties that are related to agents’ preferences and decision-making criteria (i.e., behavioural uncertainty). As agents in the model, we refer to consumers/citizens and households.
More specifically, the novelty of ATOM compared to existing models lies in obtaining realistic uncertainty bounds and splitting the total model output uncertainty in its major contributing sources, based on a variance decomposition framework and an uncertainty characterisation method, while accounting for structural uncertainty. Thus, ATOM supports the definition of uncertainty ranges, considering the type (i.e., input, parametric, and structural) and the nature of uncertainty (i.e., epistemic or aleatory), and how uncertainty propagates to the model outcomes over the planning time horizon.
ATOM is part of the Technoeconomics of Energy Systems laboratory (TEESlab) Modelling (TEEM) suite.
Key features of the ATOM model
Variance decomposition takes place for all the three main modules of ATOM (i.e., calibration, sensitivity analysis, and scenario analysis). By allowing the user to select preliminary values for the agent-related parameters according to the plausibility of its results, based on historical data and observations (goodness-of-fit statistics), the model captures input uncertainty (i.e., calibration module). By deriving forward-looking simulations for different behavioural profiles (i.e., different set of agent-related parameters), from willing to invest to risk-averse consumers, ATOM captures parametric uncertainty (i.e., scenario analysis module). Both types of uncertainty are then propagated through the model, and their contribution to the total model’s output variance is quantified. The rest uncertainty is assumed to be explained by the model’s structure. Note that the uncertainty propagation for the agent-related parameters is done for each one of them, allowing calculation of the sensitivity of each parameter to the model output, in the context of a variance-based sensitivity analysis (Sobol method), and calculation of the relative contribution of the variance for each parameter to the total model output variance (i.e., sensitivity analysis module).
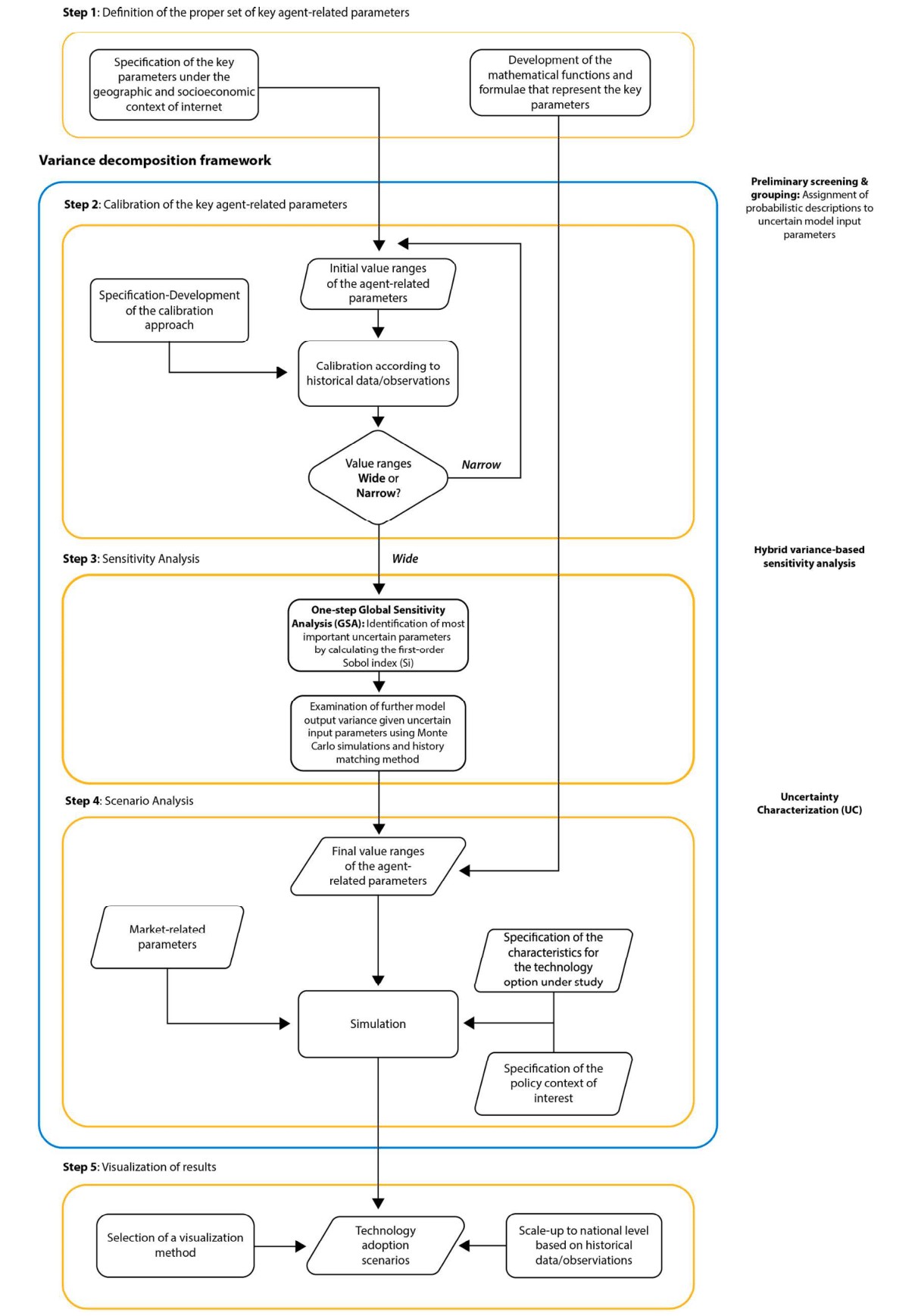
Climate module & emissions granularity
The model calculates CO2 emissions by the adoption of mitigation technologies in the residential sector, at the national scale, for the geographical and socioeconomic context under study.
Socioeconomic dimensions
Economic Growth (aspects of technology diffusion)
ATOM models the triggering of private investments, in the form of new capacity additions of different mitigation technologies, also capturing benefits and profitability aspects for households. Behavioural aspects relevant to the citizens'/households' decision-making criteria are also caputed by the model. Different socioeconomic data can be used to develop different citizen/household profiles.
Building sector growth
In ATOM, on-site energy generation and storage potential in the residential building sector is calculated, capturing also the potential socioeconomic benefits for households' self-consumption and energy autonomy.
Mitigation/adaptation measures and technologies
The model simulates the adoption of building-integrated/decentralised RES & storage technologies, energy-efficiency and adaptation measures and practices, as well as technologies and practices (e.g., demand-response, behavioural/lifestyle changes, etc.) that can increase demand-flexibility in the residential sector. It also investigates the achievement of capacity targets at the national level.
Economic rationale and model solution
The core principle of the model is that of agent-based modelling. In particular, each agent has a private initial belief about the expected annual cash inflows from investing a mitigation technology. This belief is expressed as a Gaussian distribution. Furthermore, to capture the effects of social learning, each agent receives information from the agents in its social circle that have already invested in the respective technology. This information concerns the actual profitability of their investments so far, and is used to update the agent’s beliefs. This is equivalent to updating a Gaussian prior, given a new observation. Accordingly, each agent has a social circle. This condition is modeled as a “small-world” network, which is a type of mathematical graph.
Key parameters
- Weather data (e.g., site-specific solar irradiation dataset/site-specific ambient air temperature & wind speed, etc.): Country(Space)/Hourly(Time)
- Historical data on technology adoption: Country(Space)/Daily-Monthly(Time)
- Socioeconomic data: Country(Space)/Yearly(Time)
- Electricity consumption profiles per household: Country(Space)/Hourly(Time)
- Energy prices, competitive electricity consumption tariffs, and other regulated charges: Country(Space)/Yearly(Time)
- Technological specifications: e.g., sizing of PV & storage systems, etc.
- Policy specifications: e.g., net-metering policy scheme, feen-in-tariffs, etc.
Key policies that can be addressed
Energy
- RES & storage: e.g., tax, subsidies, tariffs, etc.
- Energy efficiency: e.g., energy mix target, efficiency target, regulations (thermal regulation in buildings, banning of diesel cars in urban areas, etc.), instruments under the EED and the EPBD, performance-based financing schemes, etc.
Implications for SDGs
§7: Affordable and clean energy by investigating:
- Building-integrated/Decentralised RES generation
- Self-consumption cost-benefit ratios
- Projections on new solar PV capacity additions by households/citizens in a monthly resolution
- On-site PV energy generation and consumption projections in the residential sector
§13: Climate action by investigating:
- Adoption levels for technologies, as solar PV and storage systems, smart appliances, and electric vehicles, by households in the residential sector.
Model presentation
Video
Slides
Download slides in pdfRecent use cases
Paper DOI | Topic | Key findings |
---|---|---|
https://doi.org/10.5281/zenodo.7085526 | "Report on model application in the case studies: challenges and lessons learnt: Deliverable 7.2. Sustainable Energy Transitions Laboratory (SENTINEL) project" | Section 3.3.5.1: Empowerment of citizens' role in the energy transition is a key factor in meeting the capacity targets of small-scale PV by 2030 in Greece. Modelling outcomes showed that households' behavioural aspects play a key role in the successful roll-out of small-scale PV investments in Greece and that fostering citizens' participation in the energy system under a people-powered storyline leads to increased adoption rates and the least behavioural uncertainty related to the household’s decision-making process. They also showed that both schemes should be utilised to meet the 2030 target of 1GW of total small-scale PV capacity set by the latest version of the National Energy and Climate Plan, as the different features of each scheme may address different audiences of potential adopters. |
https://doi.org/10.1016/j.enpol.2020.111350 | A transdisciplinary modeling framework for the participatory design of dynamic adaptive policy pathways | Net-metering is capable of driving adequate investments in small-scale PV that follow the Greek PV targets’ trajectory only in the short term. This is because grid charges for net-metered electricity burden the prosumers instead of being used to increase the value of locally generated electricity for their benefit. Thus, bill savings (i.e., prosumers’ profits) are solely dependent on the electricity retail price, which reduces consumers’ willingness to install a photovoltaic system. A self-consumption scheme that subsidizes battery storage showed great potential to incentivise investments y consumers. This is because, with a self-consumption scheme prosumers consume more self-generated electricity since it can be stored for later direct use. Furthermore, grid charges are paid only for the electricity consumed from the grid. As such, there is less price-related uncertainty and prosumers’ profits are higher. However, stepwise reduction of subsidy is suggested, to ensure an initial uptake of PV, wihle avoiding excessive subsidies' cost. Regarding the applicability of AIM, the study showed that while 100% success of a policy pathway, regardless the contextual evolution, cannot be achieved, opportunities and dead-ends can be highlighted and visualized in a stakeholder-friendly fashion. |
https://doi.org/10.1016/j.apenergy.2019.113795 | An agent-based model to simulate technology adoption quantifying behavioural uncertainty of consumers | Simulation results showed that the net-metering scheme, as currently stands, is not capable of achieving the necessary capacity addition towards the Greek target of 2025. In particular, while the scheme could be successful until the end of 2021, it won’t be able to reach the national target of 2025, even in the most optimistic scenarios. The effectiveness of the net-metering scheme in Greece is closely related to the retail price of electricity. Simulation results indicated that an increase in the retail price, reduces the total uncertainty in the modeling outcomes, which is mainly introduced by modeling parameters, as the probability of investing, consumers’ beliefs and resistance towards investing. In policy terms, this is translated into a reduction of the behavioural uncertainty related to the effectiveness of the NEM scheme, especially for the case of risk-averse consumers, which implies that different sources of positive financial outcomes for consumers should be further investigated. |
References
Michas, S., Stavrakas, V., Papadelis, S., & Flamos, A. (2020). A transdisciplinary modeling framework for the participatory design of dynamic adaptive policy pathways. Energy Policy, 139, 111350. https://doi.org/10.1016/j.enpol.2020.111350.
Stavrakas, V., Papadelis, S., & Flamos, A. (2019). An agent-based model to simulate technology adoption quantifying behavioural uncertainty of consumers. Applied Energy, 255, 113795. https://doi.org/10.1016/j.apenergy.2019.113795.